Co-occurrence and diversity patterns of benthonic and planktonic communities in a shallow marine ecosystem
- 1Inmunología y Genómica, Departamento de Biotecnología y Acuicultura, Instituto de Investigaciones Marinas (IIM), Consejo Superior de Investigaciones Científicas (CSIC), Vigo, Spain
- 2Departamento de Ecología y Biología Animal, Centro de Investigación Marina (CIM), Universidad de Vigo, Vigo, Spain
- 3Department of Life Sciences, University of Trieste, Trieste, Italy
- 4Division of Oceanography, National Institute of Oceanography and Applied Geophysics (OGS), Trieste, Italy
- 5Laboratory of Marine Microbiology, Department of Earth, Environmental and Life Sciences (DISTAV), University of Genoa, Genoa, Italy
Marine microorganisms are involved in a variety of biogeochemical cycles and live in diverse ecological communities where they interact with each other and with other organisms to guarantee ecosystem functions. The present study focused on a shallow marine environment located in Ría de Vigo (NW, Spain), where sediment and size-fractionated plankton samples were collected from 2016 to 2018. DNA metabarcoding was used to describe the eukaryote and prokaryote composition and diversity in sediments and plankton and to depict possible associations among the most frequent and abundant organisms by co-occurrence network analysis. High eukaryote and prokaryote diversity indices were obtained in all compartments. Significant differences among eukaryote and prokaryote communities were found between sediment and plankton samples, with a high percentage of exclusive operational taxonomic units (OTUs) associated with each compartment, especially from sediment. Despite these differences, shared taxa between water and sediment were also obtained, suggesting a relatively meaningful exchange of organisms between both environmental compartments. Significant co-occurrences were mainly obtained between prokaryotes (41%), followed by eukaryotes–prokaryotes (32%) and between eukaryotes (27%). The abundant and strong positive correlations between organisms, including representatives from the sediment and the water column, suggested an essential role of biotic interactions as community-structuring factors in shallow waters where beneficial associations likely prevail. This study provides a novel approach for the detailed description of the eukaryote and prokaryote diversity and co-occurrence patterns in a shallow marine area, including both the sediment and different water-size fractions. The high diversity obtained and the detection of predominantly coexisting interactions among organisms from sediment and the overlying water column suggest a movement of species between both habitats and therefore confirm the importance of integratively studying shallow marine ecosystems.
1 Introduction
Aquatic microorganisms, including prokaryotes and eukaryotes, comprise the majority of ocean biomass and mediate a variety of fundamental biogeochemical cycles (Field et al., 1998; Fuhrman, 2009; Massana, 2011; Caron et al., 2012). Marine microorganisms, including autotrophs and heterotrophs, live in diverse ecological communities where they interact with each other and with viruses and multicellular organisms (Rodríguez-Martínez and Pascual, 2006; Middelboe and Brussaard, 2017; Farnelid et al., 2021; Turner et al., 2021).
Interactions between microbial species and other organisms guarantee ecosystem functions by contributing to community structure, activity, and resistance to environmental changes (Amin et al., 2012; Bjorbækmo et al., 2020; Durham, 2021; Farnelid et al., 2021);. The most common interactions include competition, mutualism, and parasitism (Amin et al., 2012; Faust and Raes, 2012; Bjorbækmo et al., 2020; Durham, 2021). Co-occurrence network analyses can assess the importance of biotic interactions (Milici et al., 2016; Brisson et al., 2019; Anderson and Harvey, 2020; Hoshino et al., 2020), which have been recognized as powerful tools to study how microorganisms are involved in the functioning of the ecosystem in sediments (Hoshino et al., 2020) and marine water (Cui et al., 2019; Hou et al., 2020). One of the most studied associations in microbial communities are those between heterotrophic bacteria and phytoplankton during microalgal bloom events (Buchan et al., 2014; Needham and Fuhrman, 2016; Zhou et al., 2018; Hou et al., 2020). These interactions have been considered essential drivers affecting key ecological processes, such as primary production or dissolved and particular organic matter (DOM and POM, respectively) remineralization by heterotrophic bacteria (Farnelid et al., 2021).
Abiotic factors such as nutrients, solar irradiation temperature, day length, and wind-driven upwelling and downwelling events influence the dynamics of the aquatic microbial community structure and induce seasonal succession of microorganisms in productive surface waters off NW Spain (e.g., Hernández-Ruiz et al., 2018; Joglar et al., 2021). These have suggested that biotic relationships may be as important as environmental variables as factors controlling the community structure in this coastal ecosystem.
Our understanding of microbial interactions in the ocean remains scarcely studied because of the reduced availability of culture strains and the high abundance of small organisms (<3 µm) in aquatic ecosystems that are not well described and play important ecological roles (Martinez-Garcia et al., 2012; Simon et al., 2015; Leray and Knowlton, 2016; Solden et al., 2016; Salazar and Sunagawa, 2017; Bjorbækmo et al., 2020). The study of the taxonomic and functional diversity of small eukaryotic and prokaryotic organisms in marine ecosystems has been growing since the application of next-generation sequencing (NGS) tools (Pavan-Kumar et al., 2015; Leray and Knowlton, 2016), circumventing the difficulties of isolating and culturing (Whittaker, 1969; Massana, 2011) and thus improving our knowledge on their ecological role in the environment (Bakker et al., 2019). Some examples of sequencing-based studies include circumnavigation initiatives, such as Malaspina or Tara Oceans, aimed at characterizing marine plankton diversity over broad geographic scales (e.g., de Vargas et al., 2015; Salazar et al., 2016), or studies more focused on smaller scales, analysing water column or sediment depth gradients or different plankton size fractions (Grattepanche et al., 2014; Gong et al., 2015; Hernández-Ruiz et al., 2018; Holman et al., 2019). Sequencing has also been widely used for describing co-occurrence patterns between microbial planktonic organisms during microalgal bloom events (Buchan et al., 2014; Needham and Fuhrman, 2016; Zhou et al., 2018; Hou et al., 2020).
The coastal ocean comprises several distinct but highly connected ecosystems that include estuaries, continental shelves, and rivers, where communities play important roles in regulating biogeochemical cycles (Bauer et al., 2013). Shallow coastal seas include some of the most dynamic marine habitats, where organisms respond quickly to human influences in the terrestrial ecosystem and interact within and between contrasting environments such as the water column and sediment (Ray, 2005; Peters and Lodge, 2009). Transport to and from the sediment and water column of microorganisms through, for example, infiltration, wind-driven mixing, tides, or bioturbation is likely to influence the diversity and function of both compartments (Garstecki et al., 2002; Forehead et al., 2013; Guizien et al., 2014; Aleklett et al., 2018, Ul-Hasan et al., 2019). While many microbial biodiversity studies have focused on the bacterial community in either sedimentary or pelagic environments, few studies have simultaneously analysed the diversity of microbial communities in shallow waters and sediments (Ul-Hasan et al., 2019).
Ría de Vigo is a highly productive system that sustains important areas of shellfish production (Fernández et al., 2016). Previous studies on microbial planktonic diversity in Ría de Vigo have mostly focused on the seasonal dynamics of a prokaryote or small (<20 μm cell size) eukaryote community in surface shelf waters (>30-m depth) (Hernando-Morales et al., 2018; Hernández-Ruiz et al., 2018) and on the description of co-occurrence networks of eukaryotic and prokaryotic associations in the epipelagic zone (Joglar et al., 2021). However, the eukaryotic and prokaryotic diversity in shallow environments (<5-m depth) within Ría de Vigo, including sediment and water samples, has not been explored thus far except for a eukaryote study by Ríos-Castro et al. (2021).
The main objectives of this study were to explore the diversity and taxonomic composition of eukaryotes and prokaryotes in sediments and plankton of a shallow area within Ría de Vigo to describe the temporal variability and the connection between the two environments and biological groups and to construct the co-occurrence network between the most abundant and frequent taxa. We hypothesize that both environments (water column and sediment) and biological groups (eukaryotes and prokaryotes) are highly connected, including a significant fraction of common and interrelated taxa.
2 Materials and methods
2.1 Sampling and DNA isolation
Eukaryotic and prokaryotic diversity was evaluated in sediment and water samples from Meira, a bivalve production area in Ría de Vigo, NW Spain (42°17′6.72″N/8°43′18.80″W) (Figure 1A). Sampling was performed approximately every 3 months from July 2016 to June 2018 (Supplementary Table 1). Superficial sediment was collected from three different areas of the intertidal zone, mixed and kept at -20°C until DNA isolation. Seawater was collected at approximately 10 m from the seashore, and it was successively filtered by different pore sizes. A volume of 75 m3 of seawater was filtered with a 200-µm plankton net to obtain the mesoplankton fraction. Subsequently, a volume of 40 l was filtered again through a 65-µm-pore-size polycarbonate filter to obtain the microplankton, and finally, 2 l of seawater was filtered using a 0.22-µm-pore-size polycarbonate filter to obtain the nanoplankton–picoplankton fraction. Filters were also kept at -20°C until DNA isolation. The nomenclature of the water fractions was adapted from Sieburth et al. (1978) (Figure 1B).
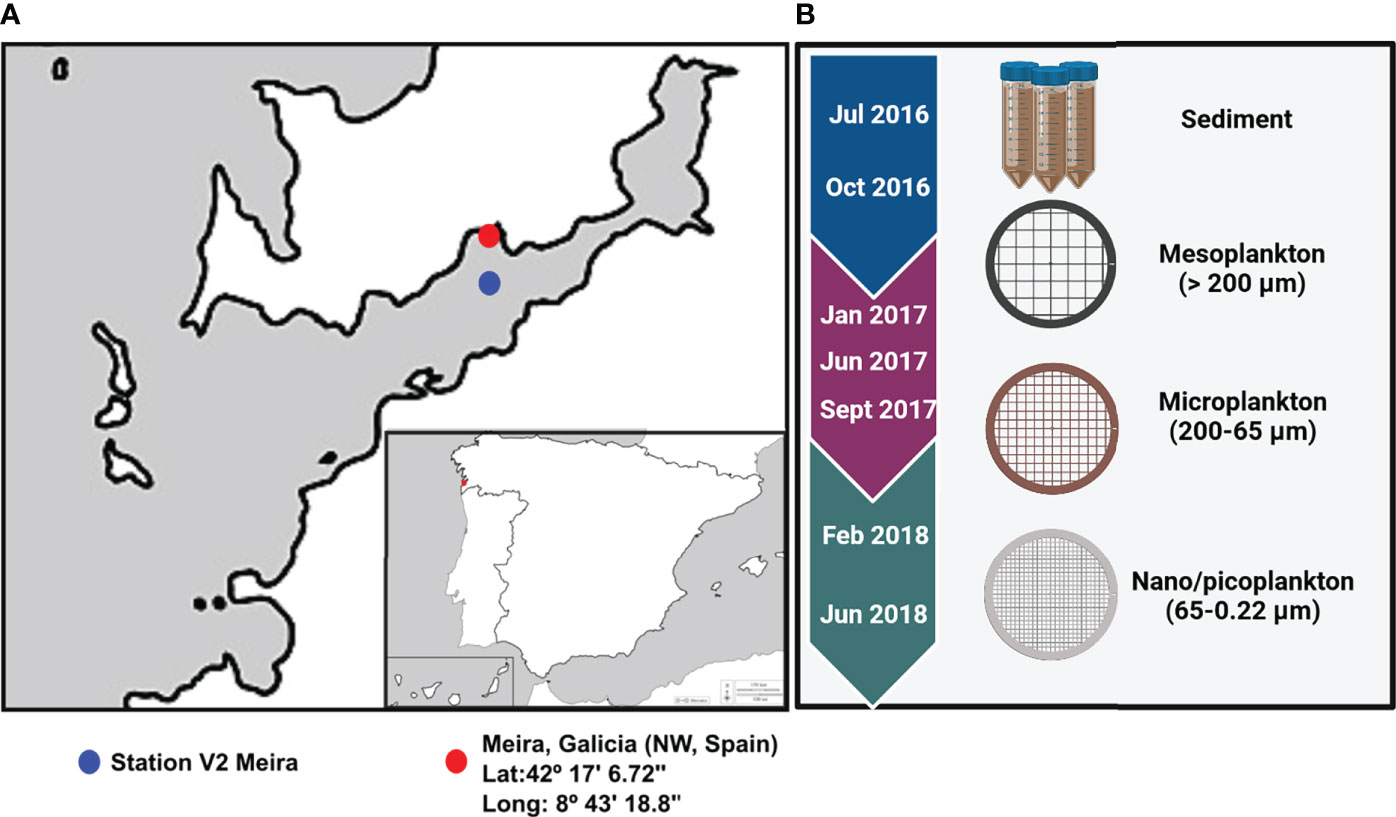
Figure 1 (A) Location of the bivalve production area in Ría of Vigo (Meira, NW Spain) and location of Station V2 where environmental parameters were obtained. (B) The sampling period extended from 2016 to 2018, and environmental samples collected for analysis were included.
A total of 28 samples of sediment and water fractions were processed. DNA from sediment and water was isolated using the PowerSoil DNA Isolation Kit (Qiagen, Düsseldorf, Germany) following the manufacturer’s protocol, and then the quality and concentration of DNA were estimated using the NanoDrop™ 1000 spectrophotometer (NanoDrop Technologies, Inc., DE, USA).
2.2 Environmental parameters
Environmental factors from the V2 station (Moaña), near our sampling site, were downloaded from Instituto Tecnolóxico para o Control do Medio Mariño de Galicia (Instituto Tecnológico para el Control del Medio Marino de Galicia (INTECMAR) and Unidad de Proceso de Datos y Sistemas de Informacion, 2010) and Meteogalicia (MeteoGalicia and Xunta de Galicia, 2022). Abiotic factors included water temperature (°C), salinity (ppm), oxygen (ml L-1), chlorophyll estimated from fluorescence (mg m-3), air temperature (°C), and precipitation (L m-2). Dissolved nutrients (µmol L-1), including NO3−, NO2−, NH4+, SiO4, HPO4-2, dissolved organic carbon (DOC), and dissolved organic nitrogen (DON), were also included in this study. The average of all surface values (0–5 m) from each of our sampling months was calculated, and values were normalized (z score) to be used in correlation Mantel test analysis (Mantel, 1967).
2.3 Amplicon sequencing and bioinformatic pipeline
The V4 region of the 16S rRNA gene (287 bp long) was amplified from 28 genomic DNA samples using the universal prokaryotic-specific primers 16S rDNA described in Lasa et al. (2019). A first target enrichment PCR assay was performed with the 16S conserved primers, followed by a second PCR assay, with customized primers including adapter complementary regions (Lasa et al., 2019). Libraries obtained from 28 environmental samples (sediment, mesoplankton, microplankton, and nanoplankton) were sequenced using an Ion Torrent (PGM) Platform (Thermo Fisher Scientific, MA). The raw read sequences obtained were deposited in the Sequence Read Archive (SRA) (http://www.ncbi.nlm.nih.gov/sra) under the BioProject accession number PRJNA828388. The bioinformatic pipeline was performed using the Microbial Genomics module (Version 1.3) of the CLC Genomics workbench (Version 9.5.1) following the protocol described in Lasa et al. (2019). Briefly, reads were quality trimmed based on quality scores and then length trimmed. Then, reads were clustered at a 97% level of similarity into operational taxonomic units (OTUs), and chimaera detection and removal were performed. Ribosomal RNA gene reads were classified against the non-redundant version of the SILVA SSU reference database (Release 123; http://www.arb-silva.de). Only reads occurring at least five times in the trimmed dataset were assigned to bacterial taxa and included in the results.
The raw eukaryote OTU table obtained after the sequencing of the V9 region of the 18S rRNA gene, performed by Ríos-Castro et al. (2021), was considered to construct co-occurrence networks of prokaryotes and eukaryotes. OTUs with a combined abundance ≤ 5 reads were considered in the present study to increase the number of nonrepresentative taxa and to be able to identify biotic and abiotic correlations of eukaryote and prokaryote communities, including not such representative taxa.
2.4 Diversity and community composition
As one of the objectives of this study was to determine the number of common OTUs between environmental compartments, the raw OTU tables of prokaryotes and eukaryotes were subsampled (Vegan R package) to the lowest number of reads per sample, which were 46,012 and 5,391 reads for eukaryotes and prokaryotes, respectively. In this way, we avoid the overrepresentation of a given compartment.
The Vegan R package was used to estimate alpha-diversity indices (Shannon–Wiener, Pielou, and OTU richness) on each sample using the subsampled eukaryote and prokaryote OTU tables. Differences in diversity indices among environmental compartments and seasons were statistically tested with the Kruskal–Wallis test and Dunn’s multiple-comparison test. The UpsetR tool (Lex et al., 2014) was used with each subsampled OTU table to estimate the number of common and exclusive eukaryote and prokaryote OTUs from each environmental compartment.
The community compositions of eukaryotes and prokaryotes were represented as relative abundances calculated from subsampled abundance OTU tables. Taxonomy was represented according to the following criteria. Overall, all orders representing >1% of the total reads were represented. When the sum of the relative abundance of all OTUs within an order was between 0.5% and 1%, these OTUs were classified at the class or phylum level. Finally, orders with abundances lower than 0.5% were grouped as “other” because of their low representativeness in the eukaryote and prokaryote composition.
2.5 Statistical analysis
To account for the inherent limitations of working with compositional data, the abundances of the subsampled OTU tables were transformed using the centred log ratio (CLR), where zero values were replaced by the minimum abundance value larger than 0 divided by 2 (Gloor et al., 2017). The CLR transformation was performed in RStudio using the Vegan R package.
Non-metric multidimensional scaling (NMDS) analysis based on Euclidean distance matrices was performed from CLR-transformed data and using the R Vegan package to study differences in eukaryote and prokaryote composition among each environmental compartment. Analysis of similarity test (ANOSIM) was used to test for differences between environmental compartments and sampling seasons. Redundancy analyses (RDA) were performed for eukaryotes and prokaryotes to analyse the association among the abiotic factors and the community composition in each environmental fraction. Permutation multiple analyses of variance (PERMANOVA) were performed to statistically explore whether the environmental parameters could influence eukaryotic or prokaryotic composition in sediment, mesoplankton, microplankton, and nanoplankton.
To statistically explore whether environmental factors could influence eukaryotic or prokaryotic composition in sediment, mesoplankton, microplankton, and nanoplankton, a correlation test was also performed (function Relate Primer3) from Euclidean distance matrices of eukaryote and prokaryote communities and the Euclidean distance matrix of environmental data (Ta water, oxygen, fluorescence, salinity, phosphate, dissolved organic carbon (DOC), and dissolved organic nitrogen (DON)). The Mantel correlation test (Mantel, 1967) was performed to calculate the correlation between eukaryotic and prokaryotic Euclidean distance matrices obtained from the CLR-transformed OTU abundance tables.
Network analyses were conducted to detect potential keystone interactions within prokaryote–prokaryote, eukaryote–eukaryote, and eukaryote–prokaryote communities. The 100 most abundant and frequent OTUs (50 eukaryotes and 50 prokaryotes) among all samples were selected to perform network analyses. To maximize the sample size, we built a single network including all sediment and water column samples together where both eukaryote and prokaryote data were available (n = 24). To avoid biases related to habitat, the CLR abundances of eukaryotes and prokaryotes were corrected by the habitat filtering (HF) algorithm (Brisson et al., 2019). Spearman correlations with the Benjamini–Hochberg p value-adjusted procedure (Benjamini and Hochberg, 1995) at a false discovery rate of 5% were conducted from HF-corrected abundances of eukaryote and prokaryote OTU tables to minimize false-positive associations. A network was built, including those significant correlations with a Spearman r > ± 0.7. The Igraph R package was used to obtain the network topology of correlations from 75 nodes and 243 significant edges (r ≥ 0.7, p < 0.05). The network obtained was compared against 100 randomized networks generated using the Erdos–Rényi model. Finally, the significance of the network was estimated by the two-sample z-test means comparing the random and real values of characteristic path length (CPL) and clustering coefficient (CL). All positive and negative significant correlations (p values ≤ 0.01) were also represented in individual networks to better visualize the connections. The environmental compartment (sediment, plankton, or both of them) on which each node was more representative was indicated in all co-occurrence networks. The following criteria were established. When the difference in relative abundance of each OTU in sediment and plankton was ≤0.5, the OTU was associated with both environmental fractions, whereas if the difference was higher than 0.5, the OTU was associated with the most representative fraction, sediment, or plankton.
3 Results
3.1 Sequencing data
A total of 28 samples for 16S rRNA sequencing were successfully amplified. A detailed description of the number of sequences obtained in each step of the analysis is specified in Table 1 and Supplementary Table 2. After sequencing, trimming, and merging prokaryote reads, a total of 906,364 reads were obtained. The prokaryote OTU-clustering process generated 9,933 OTUs obtained from 502,439 filtered reads. After the subsampling procedure performed in this study, 6,731 prokaryote OTUs were obtained and therefore considered in the following analyses (Table 1). The eukaryote sequencing results were detailed in Ríos-Castro et al. (2021). After the eukaryote OTU table subsampling procedure performed in the present study, 6,819 eukaryote OTUs were obtained and considered in the following analyses.
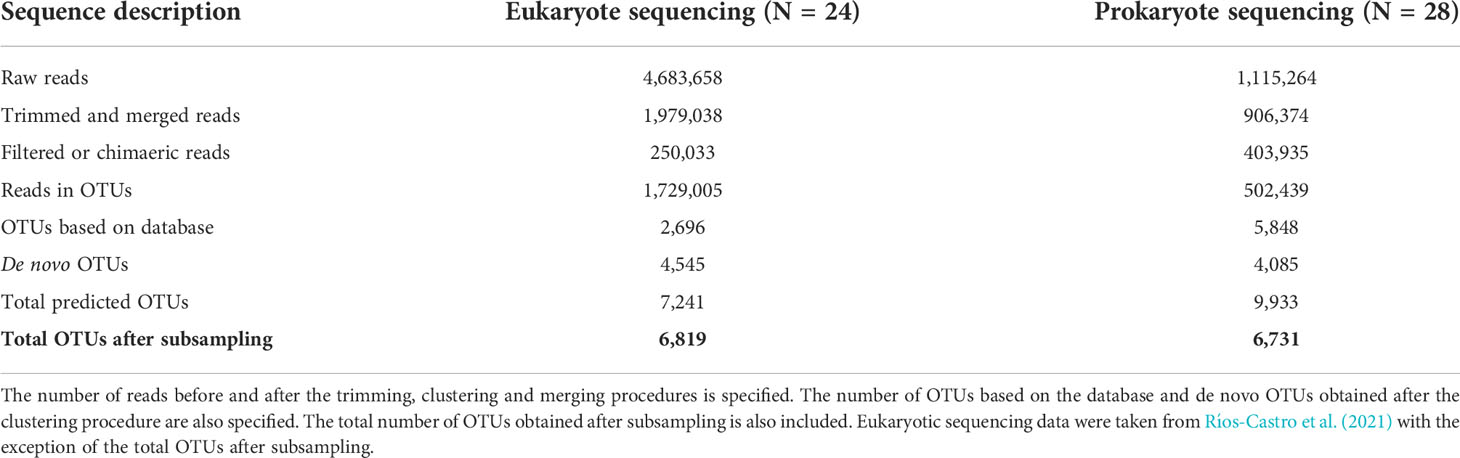
Table 1 Number of reads and OTUs obtained through the analysis of data obtained by eukaryote and prokaryote sequencing.
3.2 Environmental parameters
Abiotic parameters are represented in Figure 2. The highest rain values were recorded in February 2018, followed by June 2018 (Figure 2A). Seawater temperature followed the same patterns as air temperature, with the exception of July 2016, when the air temperature was 7°C higher than the water temperature. Compared to the other summer samplings, the low water temperature recorded in July 2016 coincided with a high upwelling index (Figure 2B).
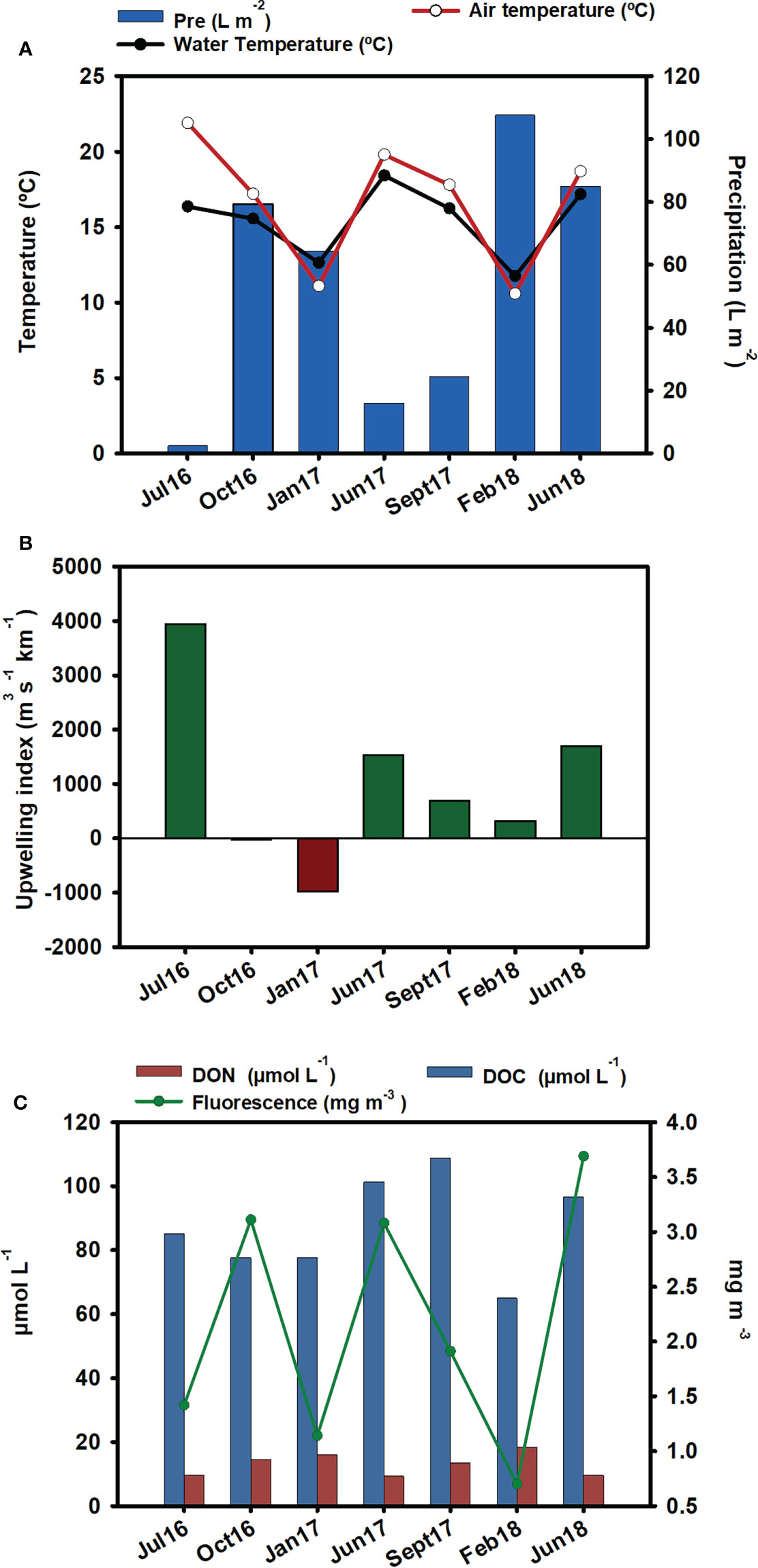
Figure 2 Environmental parameters: (A) precipitation (L m-2), water temperature (°C), and air temperature (°C). Data source: Meteogalicia (B) upwelling index. Data source: IEO. (C) DOC (µmol-L-1), DON (µmol-L-1), and fluorescence (mg m-3) from the nearest station (V2 Moaña) to our samplings. Data source: Instituto Tecnolóxico para o Control do Medio Mariño de Galicia (INTECMAR).
The lowest fluorescence values were observed in winter, especially in February 2018, which coincided with the lowest DOC value. The lowest DON was obtained in summer, and maximum values occurred in winter, mainly in February 2018. In September 2017, the highest level of DOC was observed (Figure 2C).
3.3 Overview of the eukaryote and prokaryote diversity and composition
Although eukaryotic diversity was studied previously in Ríos-Castro et al. (2021), we reanalysed the eukaryote diversity since a different and larger OTU table was considered in the present study. We also provide here a detailed description of temporal changes in the community composition, in order to link changes in the eukaryote community with those in the prokaryote community. In addition, we used here the CLR transformation of the abundance of reads to account for specific statistical constraints associated with correlation analysis.
Alpha diversity indices are specified in Supplementary Table 3. No significant differences in eukaryote richness and diversity (Shannon index) were observed among compartments (Figures 3A, B). Significant differences among sampling seasons were not observed within any of the compartments (Supplementary Figure 1). The analysis of the exclusive and common eukaryote OTUs from each environmental compartment showed that the highest number of exclusive OTUs occurred in sediment (16.3%), followed by nanoplankton (11%), microplankton (10.9%), and mesoplankton (5.7%). However, there were also a high number of OTUs common to all compartments (15.8%) (Figure 3C). The NMDS analysis of eukaryote communities showed that all sediment samples were grouped, and they were mostly separated from the other environmental compartments. The three different plankton fractions showed a minor level of separation. Mesoplankton and microplankton were mostly grouped among them, and the nanoplankton appeared more isolated from these fractions (Figure 3D). In general, significant differences were observed between environmental fractions (ANOSIM, R = 0.69, p = 0.0001). After pairwise statistical comparisons, significant differences were observed between sediment and each fraction of water (sediment-meso: ANOSIM, R = 0.95, p = 0.006; sediment-micro: ANOSIM, R = 0.83, p = 0.003; and sediment-nano: ANOSIM, R = 0.96, p = 0.006) and between mesoplankton and nanoplankton (ANOSIM, R = 0.72, p = 0.0264)
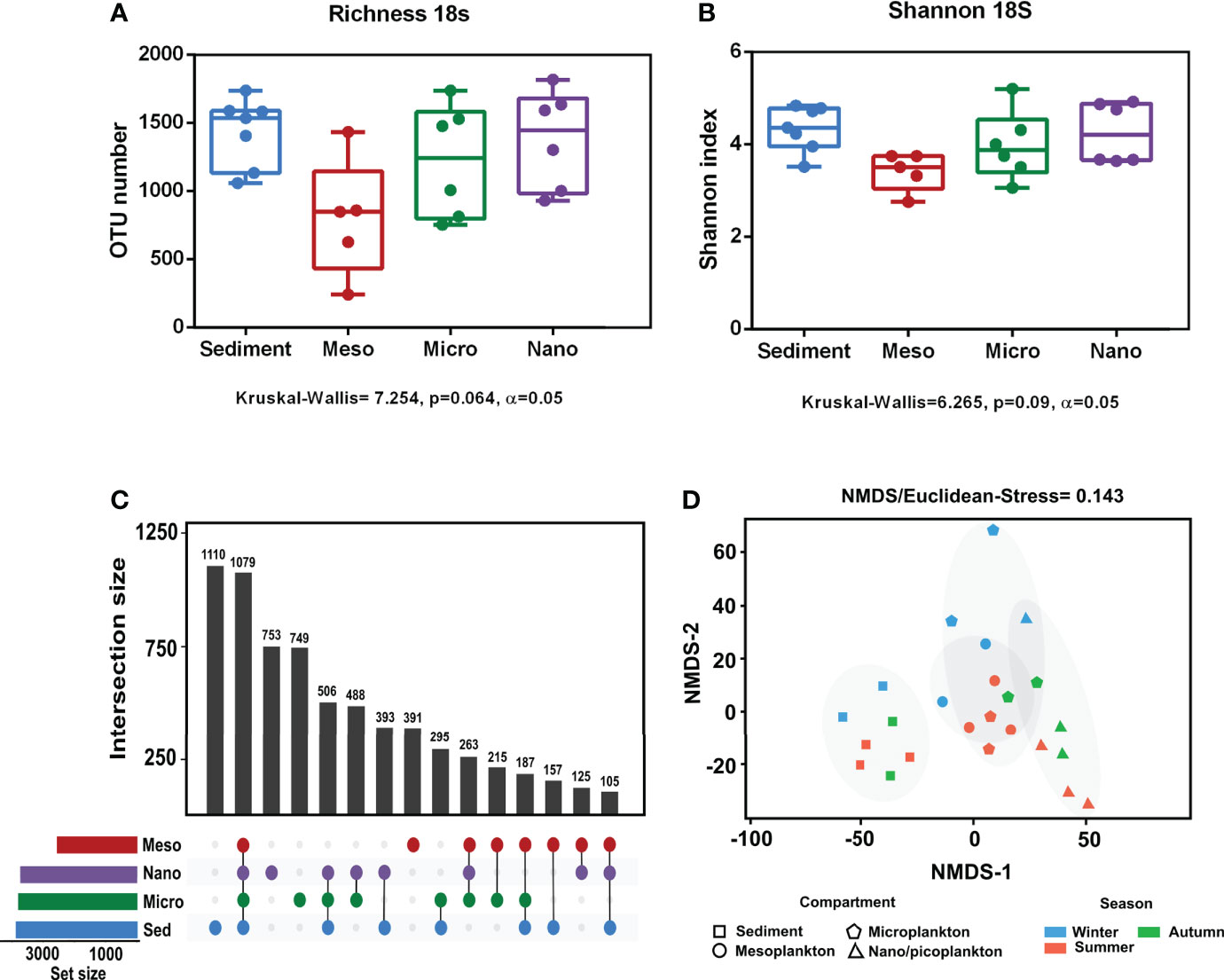
Figure 3 Alpha and beta diversity obtained from eukaryote sequencing. (A) Richness and (B) Shannon index obtained from each environmental compartment. (C) Exclusive and common OTUs of eukaryotes obtained from each environment. (D) NMDS based on Euclidean distances. The stress value indicated a reliable level of ordination.
Significant differences in prokaryote richness (Kruskal–Wallis test, p = 0.0008 < 0.001) and diversity (Shannon index) (Kruskal–Wallis test p = 0.0006 < 0.001) were observed among the environmental compartments. Significant differences were observed between sediment and nanoplankton (Dunn’s multiple-comparison test, p < 0.05) and between microplankton and nanoplankton (Dunn’s multiple-comparison test, p < 0.001) (Figures 4A, B). There were no significant differences in prokaryote richness and diversity among the sampling seasons (Supplementary Figure 1). The analysis of the exclusive and common prokaryotic OTUs showed that the highest number of exclusive OTUs was detected in sediment (17.5%), followed by microplankton (16.8%), mesoplankton (11.4%), and nanoplankton (5.6%). Compared with the high number of exclusive OTUs observed in the compartments, only 10.3% of OTUs were shared among all of them (Figure 4C). The NMDS analysis showed that samples from the same environmental compartment were clustered. The most separated clusters were sediment and nanoplankton samples (Figure 4D). Significant differences were observed between environmental fractions (ANOSIM, R = 0.75, p = 0.0001). After pairwise statistical comparisons, significant differences were observed between sediment and each water fraction (sediment-meso: ANOSIM, R = 0.77, p = 0.0054; sediment-micro: ANOSIM, R = 0.90, p = 0.002; and sediment-nano: ANOSIM, R = 1, p = 0.003), between mesoplankton and nanoplankton (ANOSIM, R = 0.518, p = 0.0048), and between microplankton and nanoplankton (ANOSIM, R = 0.897, p = 0.0042).
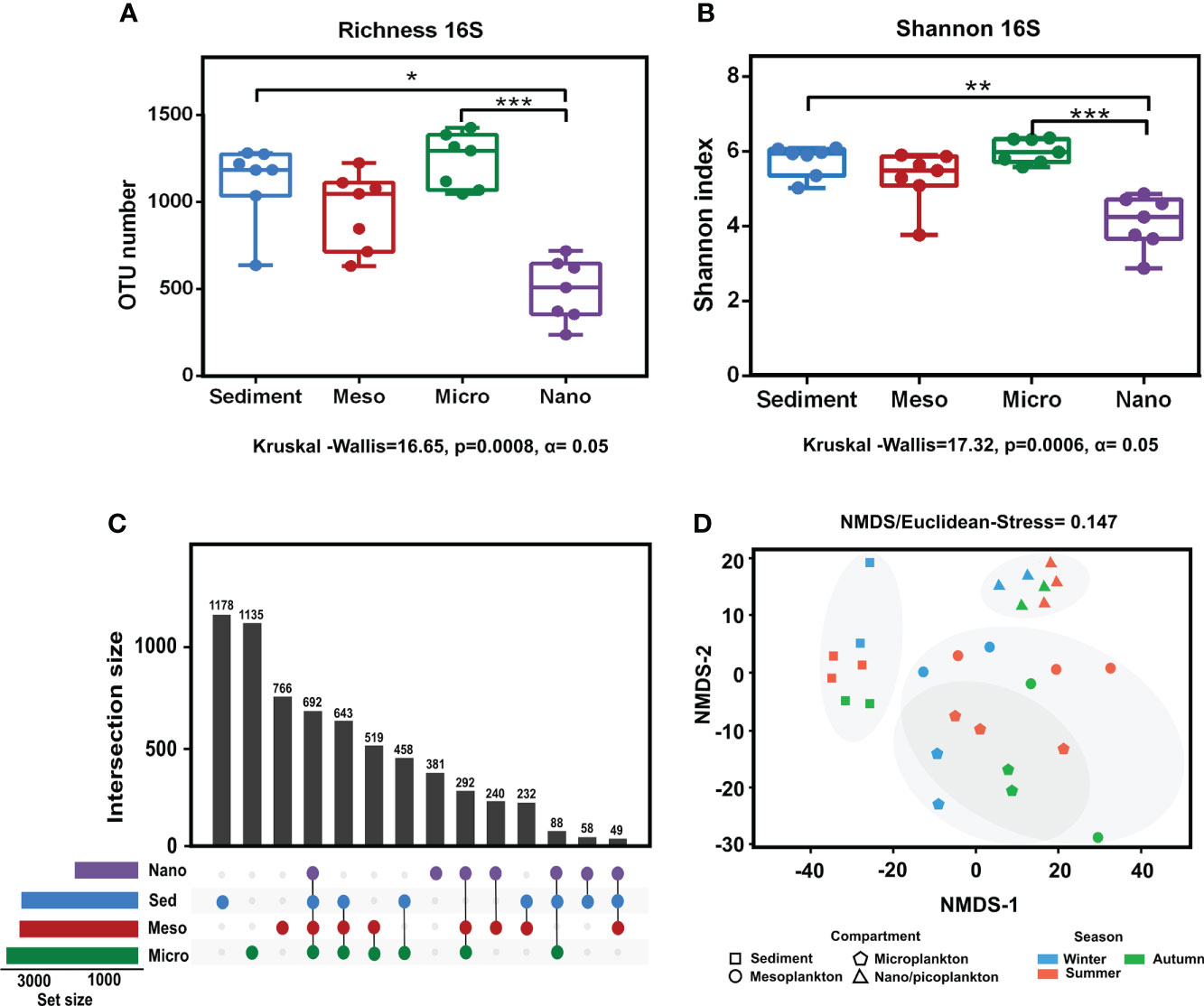
Figure 4 Alpha and beta diversity obtained from prokaryote sequencing. (A) Richness and (B) Shannon index obtained from each environmental compartment. (C) Exclusive and common OTUs of eukaryotes obtained from each environment. (D) NMDS based on Euclidean distances from each environmental compartment. The stress value indicated a reliable level of ordination. P-value < 0.05 (*); P-value < 0.01 (**); P-value < 0.001 (***).
Overall, there were no significant correlations among the environmental distance matrices and the corresponding eukaryote or prokaryote community distance matrices, except for the eukaryote community in sediments (Rho = 0.41, p = 0.048) and the prokaryote community in the nanoplankton fraction (Rho = 0.517, p = 0.009).
3.3.1 Eukaryote composition
The composition of the main eukaryote groups (Archaeplastida, Fungi, Metazoa, and Protist) present in sediment and the water column was previously described in Ríos-Castro et al. (2021), although no temporal variations were considered in each ecological fraction. In the present study, eukaryotic community composition was more deeply described.
Although the OTU tables were different, our results agree with those reported by Ríos-Castro et al. (2021), with an overall of 70%–95% of eukaryotic OTUs taxonomically classified (Figure 5). The eukaryote community greatly varied among all compartments and among the different samplings from each environmental compartment (Figure 5).
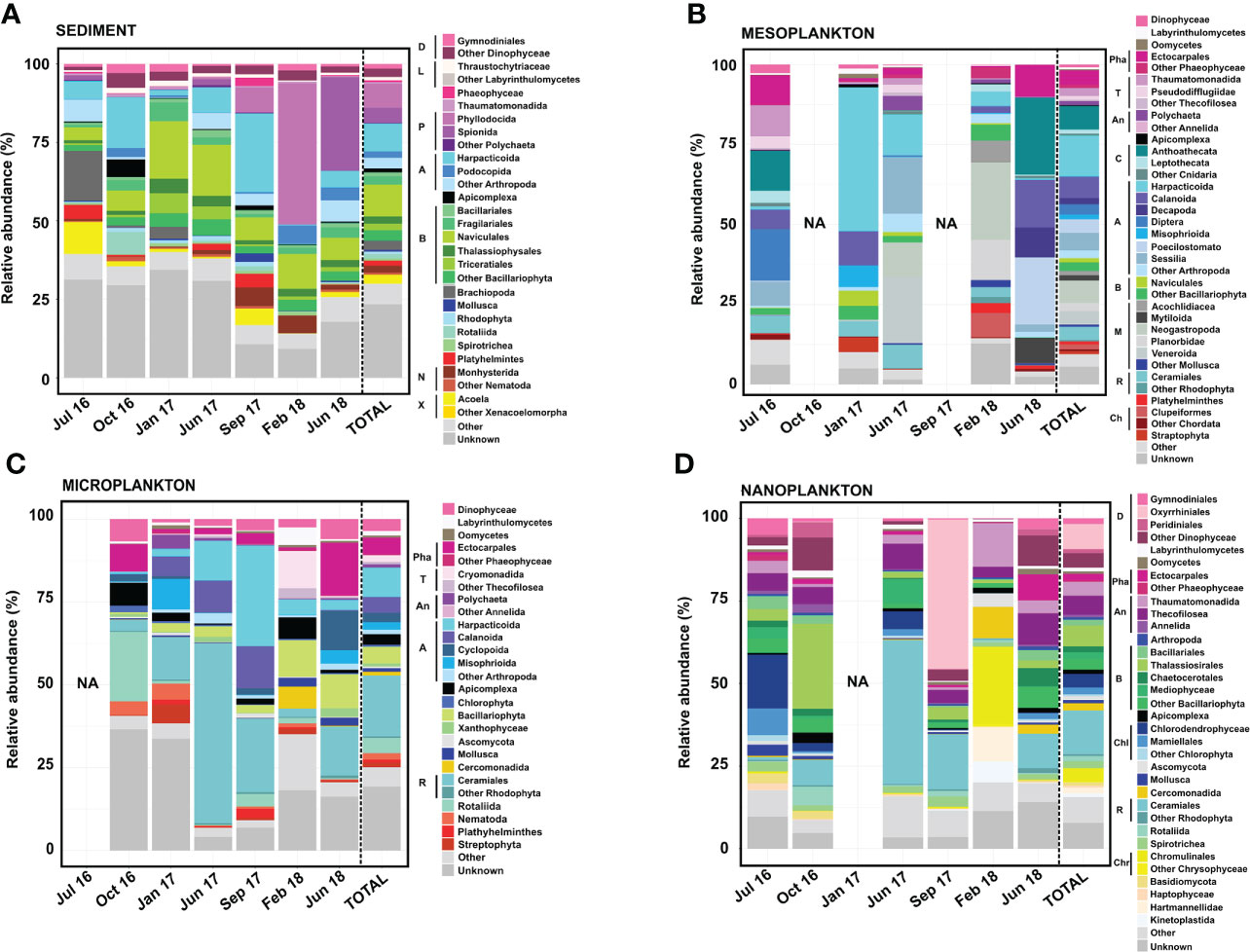
Figure 5 Eukaryote composition in terms of relative abundance obtained from (A) sediment, (B) mesoplankton, (C) microplankton, and (D) nanoplankton. Legend: A, Arthropoda; An, Annelida; B, Bacillariophyta; C, Cnidaria; Ch, Chordata; Chl, Chlorophyta; Chr, Chrysophyceae; D, Dinophyceae; L, Labyrinthulomycetes; M, Mollusca; N, Nematoda; P, Polychaeta; Pha, Phaeophyceae; R, Rhodophyta; T, Thecofilosea; and X, Xenacoelomorpha.
The Bacillariophyta phylum, Arthropoda phylum, and Polychaeta class represented the majority of eukaryote composition in sediment, with the dominance of organisms from the following orders: Naviculales (10% total reads), Harpacticoida (8.9% total reads), and Phyllodocia (7.7% total reads) (Figure 5). Massive proliferation of specific groups was eventually detected in the sediment. For example, the Polychaeta orders Phyllodocia and Spionida represented 45% of total reads in February 2018 and 30% of total reads in June 2018, respectively. Sphaerosyllis pirifera and Parapionosyllis elegans were the dominant species from the Phyllodocia bloom, whereas Pygospio elegans was the causative species of the Spionida bloom (Figure 5A).
The majority of the eukaryotic groups observed in mesoplankton corresponded with metazoans, with the phyla Arthropoda and Mollusca being the most abundant. The Harpacticoida order (Arthropoda) was the main group (13% total reads), and it accounted for 40% of the total reads in January 2017 (Figure 5B). The Mollusca phylum (18% of total reads) was mainly detected in June 2017 (32% of total reads) and February 2018 (46% of total reads), with Neogastropoda, Veneroida, and Planorbidae as the main orders (Figure 5B).
The microplankton were mainly composed of the phyla Rhodophyta (Archaeplastida) and Arthropoda (Metazoa). The order Ceramiales (Rhodophyta) contributed 18% of the total reads, and it was the dominant group in June 2017 (54% of the total reads). The order Harpacticoida was the most abundant within the Arthropoda phylum, and it was the main eukaryote taxon in September 2017 (30% of the total reads). The orders Ectocarpales and Rotaliida were particularly dominant in June 2018 (16% of total reads) and October 2016 (21% of total reads), respectively. The Bacillariophyta phylum was also detected, particularly in February and June 2018 (Figure 5C).
The majority of the eukaryotic group observed in nanoplankton corresponded with protists, with organisms from the phyla Bacillariophyta and Rhodophyta and the Dinophyceae class being the most abundant. Diatoms from the order Thalassiosirales (Bacillariophyta) were especially abundant in October 2016 (26% of total reads), while the orders Oxyrhiniales (Dinophyceae) and Ceramiales (Rhodophyta) represented 50% of total reads in September 2017 and 44% of the total reads in June 2017, respectively. Some Chlorophyta taxa were occasionally important within the nanoplankton, such as Chlorodendrophyceae and Mamiellales, which represented 16.30% and 8.07% of the total reads, respectively, in July 2016. The order Chromunilales (Chrysophyceae) was only dominant in February 2018 (Figure 5D).
3.3.2 Prokaryote composition
Almost 95% of prokaryote OTUs were taxonomically classified (Figure 6). Prokaryote composition differed among compartments, while variability among different samplings was relatively limited.
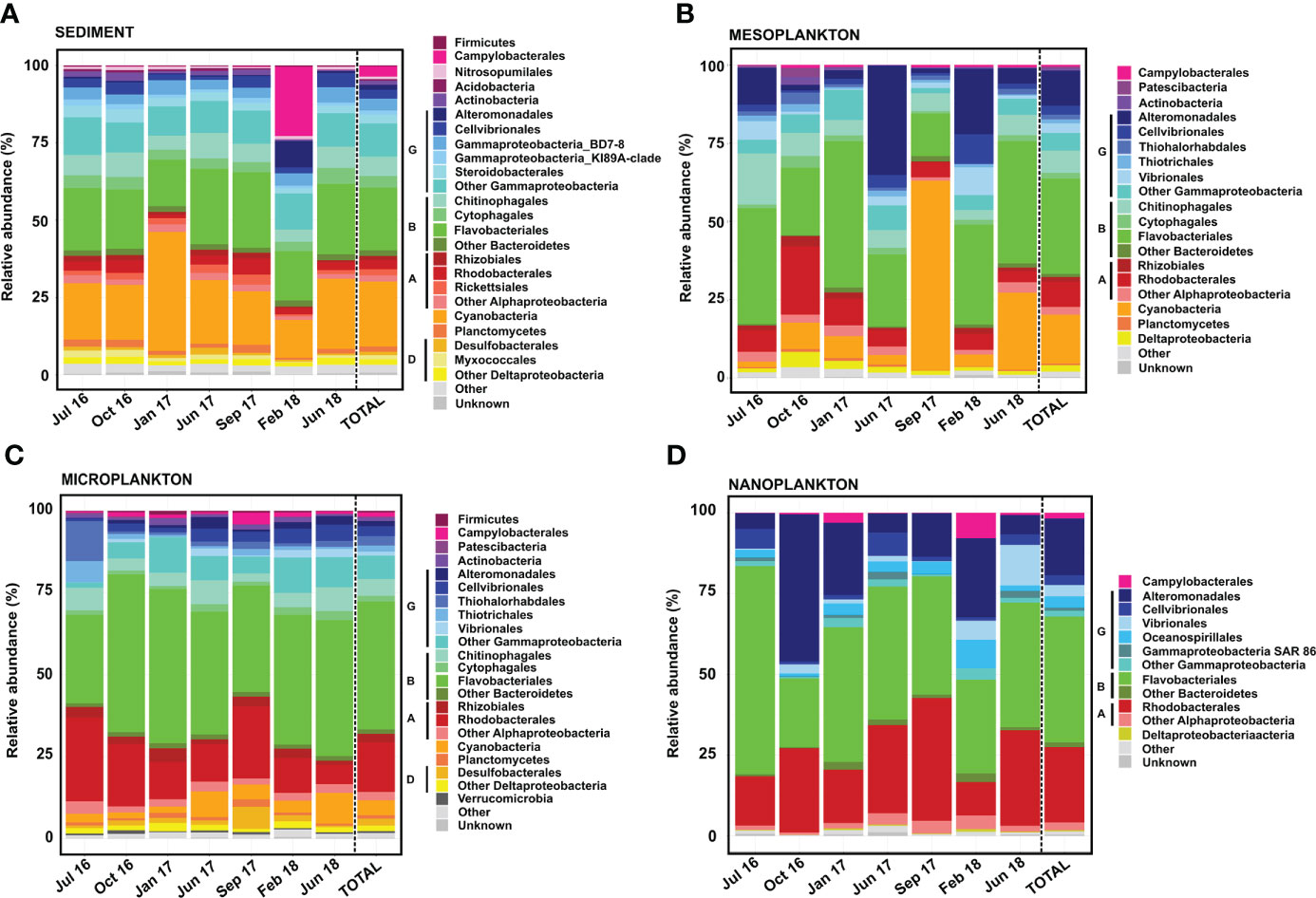
Figure 6 Prokaryote composition in terms of relative abundance obtained from (A) sediment, (B) mesoplankton, (C) microplankton, and (D) nanoplankton. A, Alphaproteobacteria; B, Bacteroidetes; D, Deltaproteobacteria; and G, Gammaproteobacteria.
Flavobacteriales, Cyanobacteria, and Gammaproteobacteria were the most abundant (21%–23% of total reads each) prokaryotes detected in sediment (Figure 6A). The Gammaproteobacteria class was composed of Alteromonadales, Cellvibrionales, Gammaproteobacteria-BD78, Gammaproteobacteria-KI8A, Steroidobacterales, and a variety of orders contributing <1% to total reads. Rhizobiales and Rhodobacterales were minor groups detected in all samplings. The prokaryote composition in sediment was relatively stable among samplings, with the exception of February 2018. In this sampling, the orders Campylobacterales and Alteromonadales represented 22% and 8% of the total reads, respectively (Figure 6A).
In the mesoplankton fraction, the order Flavobacteriales (30% total reads) and the classes Gammaproteobacteria (25% abundance) and Cyanobacteria (15% abundance) were the most representative groups (Figure 6B). In this compartment, the prokaryote composition was variable among samplings. The order Alteromonadales (Gammaproteobacteria) was mainly representative in June 2017 and February 2018 (35% and 21% total reads, respectively). The Cyanobacteria phylum represented 60% of the total reads in September 2017; however, it was not as prevalent in the other samplings. The highest contribution of the order Rhodobacterales was detected in October 2016 (22% abundance). Other groups, such as Campylobacterales, Patescibacteria, Deltaproteobacteria, and Planctomycetes, were also found in the mesoplankton (Figure 6B).
The orders Flavobacteriales and Rhodobacterales and the Gammaproteobacteria class dominated the microplankton (Figure 6C). Flavobacteriales represented 40% of the total reads and was abundant in all samplings. The Gammaproteobacteria represented 17% of the total reads, with Alteromonadales, Cellvibrionales, Thiohalorhabdales, and Vibrionales as the main orders. Cyanobacteria was also observed in all samplings but contributed less to total reads than sediment and mesoplankton compartments. In microplankton, the order Rhodobacterales represented 15% of the total reads and was detected in all samplings. Other groups contributing each <2% to total reads, such as Firmicutes, Campylobacterales, Actinobacteria, Desulfobacterales, and Verrucomicrobia, were also detected in microplankton.
The prokaryote composition of nanoplankton was similar to that of microplankton. The orders Flavobacteriales and Rhodobacterales and the Gammaproteobacteria class were also the dominant taxa; however, Cyanobacteria were not found in this compartment. The Gammaproteobacteria class was dominated by Alteromonadales, but Cellvibrionales, Vibrionales, and Oceanospirillales were also found. The prokaryote composition was more variable among samplings compared with the microplankton. Flavobacteriales represented 64% of the total reads in July 2016, whereas Alteromonadales represented 46% of the total reads in October 2016. Moreover, Campylobacterales and Oceanospirillales were more representative in February 2018, and Vibrionales was mainly detected in June 2018 (Figure 6D).
3.4 Co-occurrence networks
A strong and significant correlation was obtained between the eukaryote and prokaryote communities (Mantel test, r = 0.6324, p = 0.000999 <0.001). Significant differences were observed when the characteristic path length (CPL) and the clustering coefficient (CL) of the network, including all significant edges with rho >0.7 (Figure 7), were compared with those obtained in random networks of the same number of nodes and edges (Z-test, p < 0.001); thus, no random correlations occurred in the co-occurrence network. The topology of the co-occurrence network (Spearman r > 0.7) has been detailed in the (Table 2).
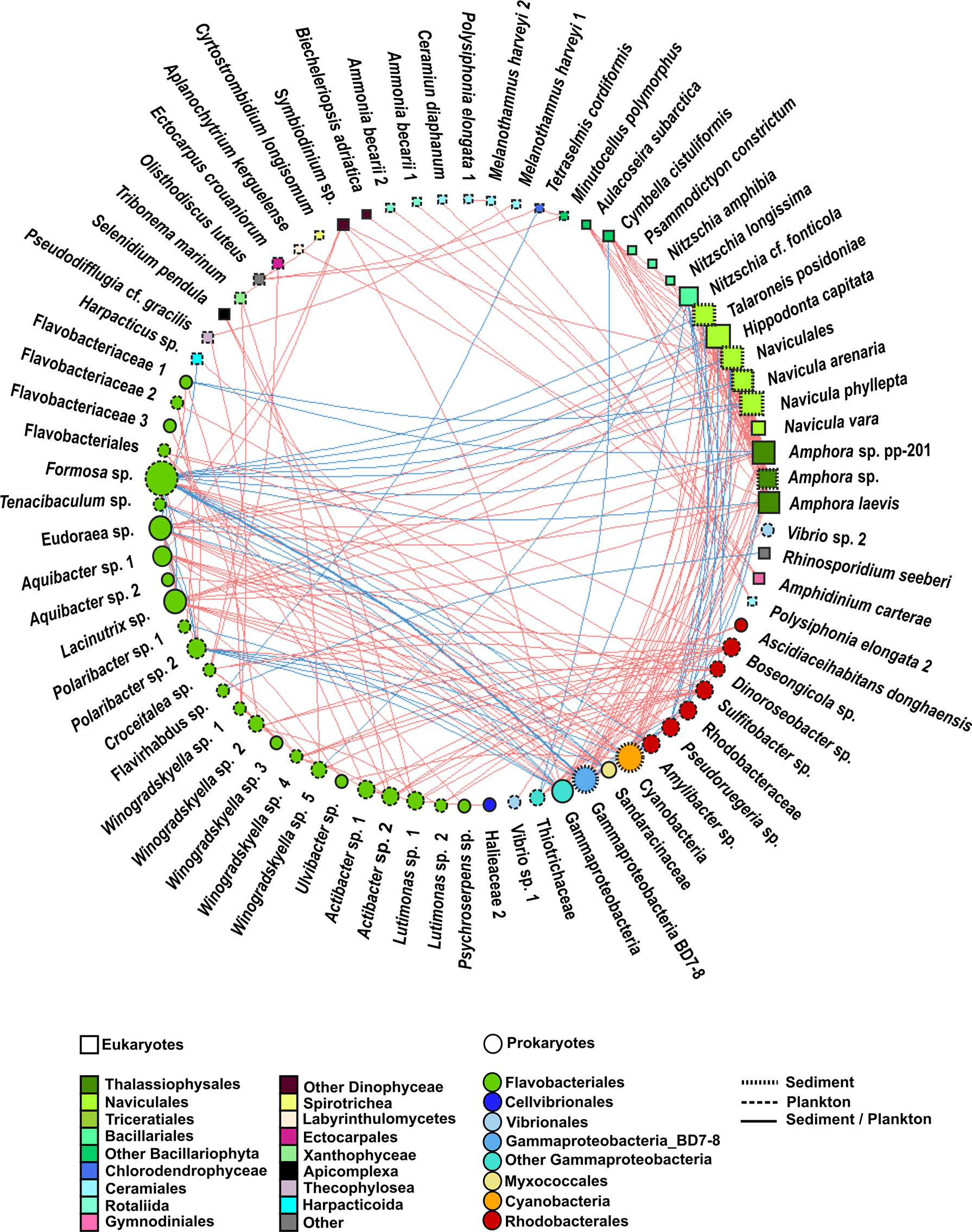
Figure 7 Undirected network that represents all the links among organisms (prokaryote–prokaryote, eukaryote–eukaryote, and eukaryote–prokaryote). Significant co-occurrences or co-exclusions (p value <0.01) were represented with a Spearman r > 0.7. Red edges represent positive correlation (co-occurrences), whereas blue edges represent negatives (co-exclusions). The size and colour of the nodes represent the degree and taxonomic group, respectively. The shape of nodes represents whether the organism is a eukaryote (square) or prokaryote (circle). The type of node lines represents the environmental compartment (sediment, plankton, or both of them) on which each OTU is more representative in terms of percentage of total prokaryotic reads.
When considering all significant edges, the majority of significant co-occurrences were between prokaryotes (41%) or between eukaryotes and prokaryotes (32%). Only 27% of the correlations occurred between eukaryotes (Figure 8). Most correlations were positive (78%). The strongest (r >0.7) positive correlations were mainly observed between eukaryotes from the Bacillariophyta phylum, whereas the strongest negative correlations involved prokaryotes (Figure 7, Supplementary Tables 4, 5). Although all the significant nodes (88 nodes) were found in sediment and plankton, 48.86% of the nodes involved in co-occurrences were mainly found in plankton, followed by 42.04% of nodes which showed a similar relative abundance in both sediment and plankton fractions. Despite that only 9.09% of total OTUs were mainly associated with sediment samples (mainly diatoms), they were highly correlated with eukaryotes and prokaryotes from both environments.
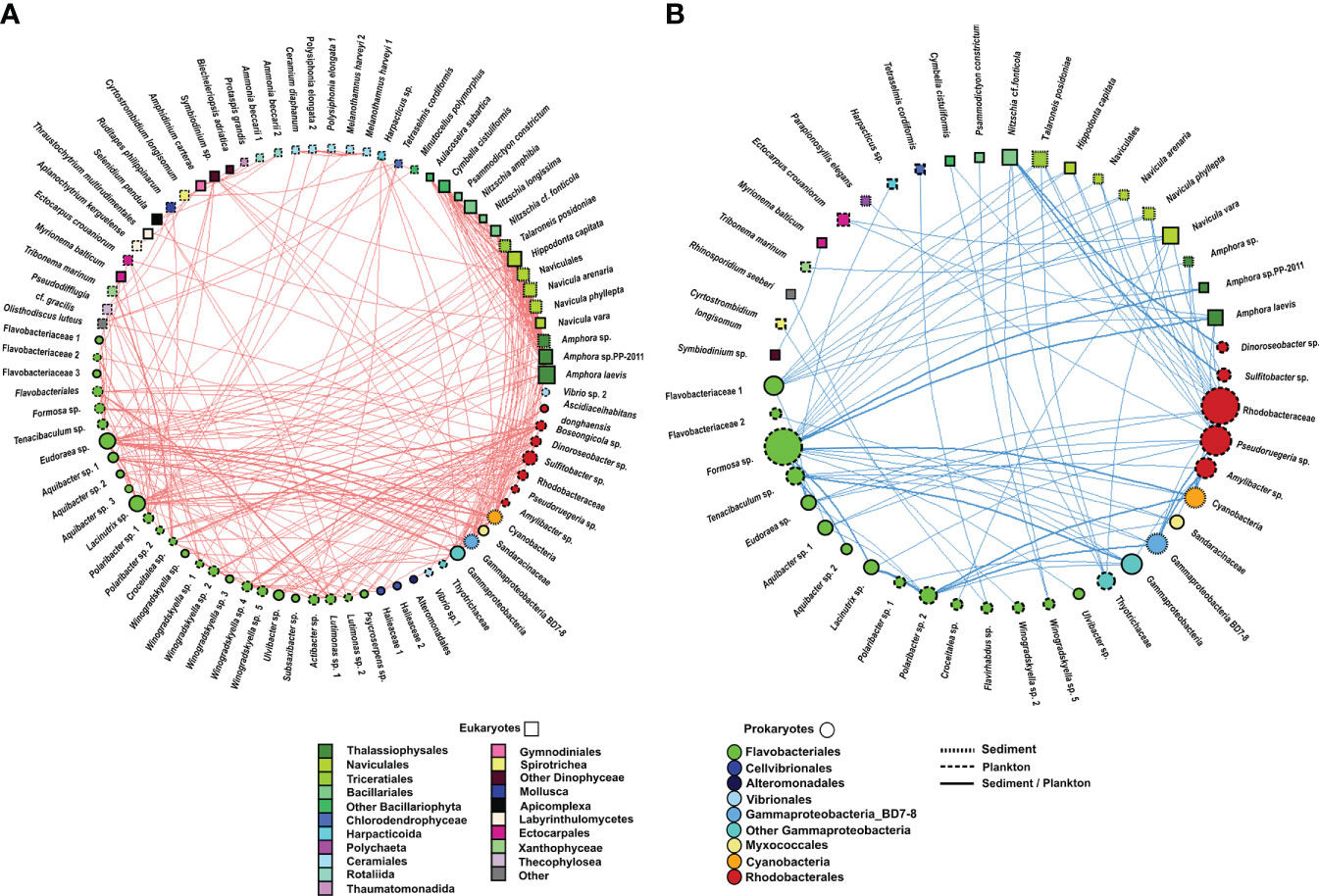
Figure 8 Undirected network that represents all links among organisms (prokaryote–prokaryote, eukaryote–eukaryote, and eukaryote–prokaryote). All significant correlations (p value <0.01) were represented. (A) Positive correlations and (B) negative correlations. The size and colour of the nodes represent the degree and taxonomic group, respectively. The shape of nodes represents whether the organism is a eukaryote (square) or prokaryote (circle). The type of node lines represents the environmental compartment (sediment, plankton, or both of them) on which each OTU is more representative in terms of percentage of total prokaryotic reads.
3.4.1 Prokaryote–prokaryote correlations (rho >0.7)
Only 10.29% of correlations were negative among prokaryotes. Most correlations among prokaryotes included OTUs from the Flavobacteriales order (31%), followed by correlations involving the Flavobacteriales and Rhodobacterales orders (26%) and those including members of the Flavobacteriales order and the Gammaproteobacteria class (14%) (Figure 7, Supplementary Table 4, Supplementary Figure 2). However, the strongest positive and negative correlations were observed between the pairs Gammaproteobacteria-BD7-8 and Cyanobacteria and Gammaproteobacteria-BD7-8 and Formosa sp., respectively. Furthermore, correlations between Flavobacteriales genera, such as Eudoraea sp.-Lacinutrix sp. and Eudoraea sp.- Aquibacter sp., were among the strongest positive bacterial relations, and they were also included among the strongest negative correlations with Gammaproteobacteria-BD7-8 (Figure 7, Supplementary Table 4).
Myxococcales were involved in weaker correlations with Rhodobacterales, Cyanobacteria, and Gammaproteobacteria, each one contributing less than 1% of bacterial co-occurrences (Supplementary Figure 2).
3.4.2 Eukaryote–eukaryote correlations (rho >0.7)
All eukaryote correlations with a Spearman rho >0.7 were positive (Figure 7). Positive correlations mainly occurred between benthic diatoms (80%) from the following orders: Naviculales (including the genera Hippodonta and Navicula), Thalassiophysales (genus Amphora), Triceratiales (genus Talaroneis), and Bacillariales (genus Nitzschia) (Figure 7, Supplementary Table 4, Supplementary Figure 2).
Weaker correlations were also found between pairs of OTUs from the order Ceramiales (Polysiphonia elongata with Melanothamnus harveyi and Ceramium diaphanum), between Dinophyceae and Rhizaria species (Amphidinium carterae-Ammonia beccarii), and between Dinophyceae and Bacillariophyta (Symbiodinium sp.- Amphora sp.) (Figure 7, Supplementary Table 4).
3.4.3 Eukaryote–prokaryote correlations (rho >0.7)
Eukaryote–prokaryote correlations mainly involved eukaryotes from the Bacillariophyta phylum and the following prokaryotes: Flavobacteriales (31%), Gammaproteobacteria (23%), Rhodobacterales (14%), and Cyanobacteria (12%) (Figure 7, Supplementary Table 4, Supplementary Figure 2).
Positive and negative correlations between Bacillariophyta and Flavobacteriales were observed. For example, positive relations were observed among the Eudoraea and Lacinutrix genera with the diatoms Hippodonta capitata, Naviculales and Amphora sp. Most negative correlations occurred between Formosa sp. (Flavobacteriales) and the diatoms Navicula sp., Hippodonta capitata, and Amphora sp. The correlations among the diatoms Hippodonta sp. and Naviculales with Gammaproteobacteria and Cyanobacteria were also positive, whereas a negative relation was detected among the diatom Nitzschia sp. and a Gammaproteobacteria from the Thiotrichaceae family. All correlations among Bacillariophyta and Rhodobacterales (including the genera Pseudoruegeria, Dinoroseobacter, or Sulfitobater) were negative. The strongest negative association was detected between Sulfitobacter sp. and Nitzschia cf. fonticola. Other positive correlations involved Flavobacteriales with Dinophyceae (Symbiodinium sp.), Xanthophyceae (Tribonema sp.), or Apicomplexa (Selenidium pendula). A negative correlation involving Flavobacteriales and Arthropoda (Harpacticus sp.) was also observed. The association between Harpacticus sp. and Tenacibaculum sp. (Flavobacteriales) was negative (Figure 7, Supplementary Table 4).
4 Discussion
A major novelty of this study is that it provides the first detailed description of the eukaryote and prokaryote co-occurrence network in a shallow (<5-m depth) marine area near the intertidal zone in Ría de Vigo, including both the sediment and water column (meso- to picoplankton). The eukaryotic composition was previously studied in the same area by Ríos-Castro et al. (2021). A wide variety of organisms move across different ecosystems throughout their life cycles, using these habitats as spawning sites, refuges, nurseries, or feeding grounds (Levin et al., 2001). The movement of organisms between the benthic and pelagic realms makes shallow seas highly diverse ecosystems with increasing richness toward the shoreline (O'Donnell et al., 2017; Sánchez-Soto Jiménez et al., 2018). Previous metabarcoding studies conducted in Ría de Vigo focused on the study of eukaryote and/or prokaryote communities in deeper areas (>30 m depth) and involved mostly the micro- to picoplankton size fractions (Hernando-Morales et al., 2018; Hernández-Ruiz et al., 2018; Joglar et al., 2021; Gutiérrez-Barral et al., 2021). Although not directly comparable due to the inclusion of different sample sizes and the use of different primer sets, the number of unique OTUs was overall higher in the present study than in these previous studies in deeper areas within Ría de Vigo or the adjacent shelf (Hernández-Ruiz et al., 2018; Joglar et al., 2021; Gutiérrez-Barral et al., 2021), which highlights the importance of studying eukaryote and prokaryote communities in shallow waters, where the high diversity can be related to the proximity of sediment communities. The percentage of water-sediment shared taxa (39.91% for eukaryotes and 34.84% for prokaryotes) suggests a relevant exchange of taxa between both habitats. A similar percentage of shared taxa among sediment and the overlying water column was reported for another shallow marine environment (e.g., Chen et al., 2017). Several studies have suggested that sediments should be considered as seed banks of planktonic diversity (Itakura et al., 1997; Massana et al., 2015; Likumahua et al., 2021), while propagules and larvae from benthic organisms can swim in the overlying water column. Our dataset is consistent with this picture, as abundant organisms in planktonic fractions, such as Dinophyceae or Ceramiales, were observed in low abundance in sediments. A co-occurrence network showed a high percentage of OTUs with a similar abundance in both sediment and plankton, some of which were involved in associations with both benthonic and planktonic organisms, like for example, the positive association of the bacteria Eudoraea sp. (found in both compartments) with benthic Cyanobacteria and diatoms (e.g., Naviculales), or the positive association of the apicomplexan Selenidium pendula with several planktonic Flavobacteriales. Furthermore, strong correlations among mostly planktonic and mostly benthonic organisms were also found in co-occurrence networks, like for example the co-exclusions found between Formosa sp. (plankton) and Gammaproteobacteria BD7-8 (sediment) and benthic diatoms. These associations reinforce the idea of a relevant connection between sediment and plankton taxa and the importance of including both compartments in the study.
Previous studies performed in the open ocean suggested that the highest eukaryote diversity occurs in the smallest size fraction (de Vargas et al., 2015; Leray and Knowlton, 2016). The same pattern was observed in our study for eukaryote richness as well as in Ríos-Castro et al. (2021). In contrast, lower richness and diversity of prokaryotes was found in the smaller plankton size fractions, as previously reported (e.g., Mestre et al., 2017). On average, a higher diversity of both eukaryotes and prokaryotes was found in sediments than in water samples, as previously observed in other marine ecosystems (Probandt et al., 2017; Ul-Hasan et al., 2019; Zhao et al., 2020). Such patterns are likely related to distinct benthic environments associated with sharp gradients in physicochemical factors. The significant differences found between taxonomic composition between sediment and plankton samples and the high percentage of exclusive OTUs found in sediment may be related to the unique conditions within sediments compared with the water column realm (Zinger et al., 2011).
Independent of the differences observed among compartments, eukaryote composition showed higher temporal variability than prokaryote composition. Moreover, the higher distances found between eukaryotes than between prokaryote samples in the NMDS reinforce the idea of a higher dynamism of eukaryotes compared to prokaryotes. In general, prokaryote composition was homogeneous between samplings from the same compartment. The punctual presence of the pathogen Campylobacter sp. February 2018 in sediment could be explained by faecal contamination (Perkins et al., 2014) produced in the zone near a wastewater treatment plant.
The highest proportion of unknown organisms was obtained from eukaryotes in sediment and microplankton samples, while almost 98% of prokaryotes were taxonomically classified. Previous studies suggested that the wide number of unknown organisms present in sediment belongs to the protist group (Forster et al., 2016), as the majority of small eukaryotes (<20 µm cell size) (24% to 98%) remain to be described (Leray and Knowlton, 2016). The larger fraction of undescribed eukaryotes compared to prokaryotes could be related to the fact that the first metabarcoding studies mainly focused on bacteria. Consequently, prokaryote reference databases might be more complete. Although DNA metabarcoding has allowed the characterization of new eukaryote taxa in recent years, there are still many unknown protists not available in public reference datasets.
Interactions between organisms contribute to the structure and function of the community and ecosystem. Although previous studies reported that abiotic factors strongly influence the dynamics of the community (Liu et al., 2021; Yu et al., 2022), others indicate that biotic interactions also play an important role (Steele et al., 2011; Gilbert et al., 2012; Chow et al., 2013). The strong correlation between organisms and the low explanatory capacity of abiotic factors for community composition suggest an essential role of biotic interactions as community-structuring factors in shallow waters, as previously proposed for planktonic communities in surface waters of Ría de Vigo (Hernández-Ruiz et al., 2018; Joglar et al., 2021). Nevertheless, the role of environmental factors in the present study may be underestimated, particularly for ambient sediment.
Overall, positive correlations, reflecting significant co-occurrences among OTUs, clearly prevailed in these shallow waters, suggesting that mutualistic and cooperative interactions are important in the study area. The percentage of co-occurrences was higher than in surface waters of Ría de Vigo (Hernández-Ruiz et al., 2018; Joglar et al., 2021). Several studies have suggested that facilitation interactions might favour coexistence (Brooker et al., 2008; Cavieres and Badano, 2009), which is also consistent with the higher diversity found in shallow sampling sites (this study) compared to previous deeper sampling sites in Ría de Vigo. The interactions between diatoms and bacteria have been extensively studied in recent years (Amin et al., 2012; Zhou et al., 2018; Hou et al., 2020) and were frequent in our study, clearly dominating the eukaryote–prokaryote co-occurrences.
Positive correlations could be explained by cross-feeding, co-colonization, niche overlap, or co-aggregation in biofilms (Faust and Raes, 2012). Therefore, the strong positive correlation among heterotrophic bacteria from the Flavobacteriales order, mainly found in planktonic fraction, might reflect mutualist or cooperative interactions. The strongest positive correlation between the heterotrophic benthic Gammaproteobacteria-BD7-8 (Li et al., 1999) and the autotrophic Cyanobacteria, also representative in sediments, could be explained by a mutualist interaction produced in this environmental compartment, involving the remineralization of DOC (produced by Cyanobacteria during photosynthesis) by the bacteria (Gammaproteobacteria), which would subsequently supply inorganic nutrients to cyanobacteria. Although few negative correlations were observed among bacteria, they were probably caused by a competition for limiting resources (Faust and Raes, 2012).
Protist representatives from the TSAR (Telonemida, Stramenopiles, Alveolata, and Rhizaria) supergroup were dominant in the networks (86% of the significant eukaryote nodes), which agrees with their great abundance and the variety of crucial roles they play in the marine environment (Burki et al., 2021).
Correlations among eukaryotes were mainly positive (only four among 105 eukaryote–eukaryote correlations were negative), suggesting that the competition among eukaryotes is minimal in comparison with that within bacteria. Interestingly, the most robust positive correlations occurred among benthic diatoms (TSAR). The strong association between benthic diatoms could be related to a cooperative strategy to optimize resource exploitation through cross-feeding and facilitation events. Several studies confirmed that benthic diatoms from different species, such as Amphora sp. or Navicula sp. (Molino and Wetherbee, 2008), establish cooperative relations within benthic biofilms, which might increase biomass production (Loreau and Hector, 2001; Koedooder et al., 2019) and counteract harsh limiting conditions that occur in estuarine and intertidal zones, such as desiccation, salinity, and temperature variations (Vanelslander et al., 2009).
Diatoms were also involved in the majority of eukaryote–prokaryote co-occurrences. They take up inorganic CO2 in benthic and pelagic areas and release dissolved and particular organic matter (DOM and POM, respectively), which promotes the growth and remineralization activity of heterotrophic bacteria that in turn supply inorganic nutrients necessary for diatom growth (Cole, 1982; Serôdio and Lavaud, 2020; Zheng et al., 2020; Farnelid et al., 2021). A wide number of studies have demonstrated that the main heterotrophic phyla associated with diatoms are Bacteroidetes (Flavobacterium sp.) and Proteobacteria (Sulfitobacter sp., Alteromonas sp., and Roseobacter sp.) (Grossart et al., 2005; Sapp et al., 2007; Amin et al., 2012). In the present study, associations between eukaryotes and prokaryotes involved different benthic diatoms and heterotrophic bacteria from the Flavobacteriales order, which were mainly found in the planktonic fraction. Co-occurrence patterns among diatoms and bacteria are typically explained by the mutualist interaction encompassing organic matter, including growth factors, and inorganic nutrient exchange (Amin et al., 2012). Therefore, our data suggest that the metabolite trading between diatoms and bacteria may also occur between the water column and the sediment in shallow areas. Positive correlations between diatoms and Rhodobacterales could also be explained by the bacteria supplying limiting growth factors such as vitamin B12 and B1 (Croft et al., 2005; Luria et al., 2016; Joglar et al., 2021). On the other hand, antagonistic associations were mainly found between Flavobacteriales (mainly Formosa sp.) and Rhodobacterales with diatoms. Such negative associations could be mediated by the production of antibacterial compounds by the diatoms, such as fatty acids, that affect bacterial growth (Wichard et al., 2007; Amin et al., 2012), by the production of algaecides by bacteria, or by a pH increase as the result of bacteria NH+4 or H2S oxidation, which inhibit algal growth (Cole, 1982; Stock et al., 2019). Tenacibaculum sp. was detected in all planktonic compartments, especially in nanoplankton, and was negatively associated with arthropods from the order Harpacticoida also detected in planktonic fraction, suggesting a possible pathogenic interaction. In Spain, Tenacibaculum maritimum has caused tenacibaculosis disease with important economic losses in the culture of commercial fishes such as Scophthalmus maximus, Salmo salar, Solea senegalensis, and Sparus aurata (Fernández-Álvarez and Santos, 2018). This organism is a pathogen of commercial fishes; however, it was also isolated from shrimp (Arthropoda), causing cotton shrimp-like disease (Sheu et al., 2007; Zhou et al., 2019).
5 Conclusion
In conclusion, the use of the metabarcoding methodology based on the sequencing of the V9 and V3–V4 regions of the 18S rRNA and 16S rRNA genes, respectively, has provided valuable information on the eukaryotic and prokaryotic composition of sediment and planktonic fractions. Despite the recent interest in including sediment in metabarcoding studies (Forster et al., 2016; Holman et al., 2019), few studies have been conducted in shallow-water communities in sediment and the water column. In the present study, it was possible to highlight the higher diversity obtained in the shallow area (<5-m depth) compared to previous studies in deeper zones of Ría de Vigo. This could be strongly related to the exchange of organisms between the benthic and pelagic zones, the large diversity associated with sediments, and the existence of a wide number of co-occurrences between organisms that could favour their coexistence. All these facts suggest the importance of following integrative approaches to study shallow marine ecosystems, which are also highly influenced by anthropogenic factors, such as the introduction of foreign species by ballast water or the release of pollutants to the marine ecosystem (Pecl et al., 2017; Veilleux et al., 2021). In particular, Ría de Vigo is characterized by its high productivity in the aquaculture sector and its exposure to several anthropogenic factors derived from industrial and domestic wastes (Guerra et al., 2002). Our study also shows that metabarcoding approaches could be useful tools for the integrative and systematic monitoring of diversity as well as for detecting potential biotic interactions among organisms in shallow marine environments, which are some of the most endangered areas in the context of global change.
Data availability statement
The datasets presented in this study can be found in online repositories. The names of the repository/repositories and accession number(s) can be found below: https://www.ncbi.nlm.nih.gov/, PRJNA828388.
Author contributions
RR-C: methodology, formal analysis, investigation, data curation writing—original draft, and visualization. CC-S: methodology, formal analysis, data curation, visualization, and review and editing. AP: software, formal analysis, data curation, and writing—review and editing. LV: software, formal analysis, data curation, and writing—review and editing. BN: conceptualization, resources, methodology, writing—review and editing, supervision, and funding acquisition. ET: conceptualization, methodology, formal analysis, data curation, writing—review and editing, and supervision. AF: conceptualization, resources, methodology, formal analysis, writing—review and editing, supervision, and funding acquisition. All authors contributed to the article and approved the submitted version.
Funding
This work was supported by the Ministerio de Economía y Competitividad, Spain (CTM2017-83362-R), Consellería de Economía, Emprego e Industria–GAIN, Xunta de Galicia (IN607B 2019/01), Fondo Europeo de Desarrollo Regional FEDER en el marco del programa Interreg V A España – Portugal (POCTEP) 2014-(20200474_BLUEBIOLAB), VIVALDI [678589] (EU H2020), and Controlling Microbiomes Circulations for Better Food Systems” (CIRCLES) [818290] (EU H2020). RR-C wishes to thank the Axencia Galega de Innovación (GAIN, Xunta de Galicia) for her predoctoral contract (IN606A-2018/020).
Acknowledgments
We want to thank Raquel Aranguren and Begoña Villaverde for their help with sampling. We want to thank Elisa Banchi for bioinformatics help.
Conflict of interest
The authors declare that the research was conducted in the absence of any commercial or financial relationships that could be construed as a potential conflict of interest.
Publisher’s note
All claims expressed in this article are solely those of the authors and do not necessarily represent those of their affiliated organizations, or those of the publisher, the editors and the reviewers. Any product that may be evaluated in this article, or claim that may be made by its manufacturer, is not guaranteed or endorsed by the publisher.
Supplementary material
The Supplementary Material for this article can be found online at: https://www.frontiersin.org/articles/10.3389/fmars.2022.934976/full#supplementary-material
Abbreviations
OTU, operational taxonomic units; DOM, dissolved organic matter; POM, particular organic matter; NGS, next-generation sequencing; SRA, Sequence Read Archive; DON, dissolved organic nitrogen; CLR, centered log-ratio; NMDS, non-metric multidimensional scaling; RDA, redundancy analysis; PERMANOVA, Permutation Multiple Analyses of the Variance; HF, habitat filtering; CPL, characteristic path length; CL, clustering coefficient; and ANOSIM, analysis of similarities.
References
Aleklett K., Kiers E. T., Ohlsson P., Shimizu T. S., Caldas V. E., Hammer E. C. (2018). Build your own soil: exploring microfluidics to create microbial habitat structures. ISME J. 12 (2), 312–319. doi: 10.1038/ismej.2017.184
Amin S. A., Parker M. S., Armbrust E. V. (2012). Interactions between diatoms and bacteria. Microbiol. Mol. Biol. Rev. 76 (3), 667–684. doi: 10.1128/MMBR.00007-12
Anderson S. R., Harvey E. L. (2020). Temporal variability and ecological interactions of parasitic marine syndiniales in coastal protist communities. mSphere 5 (3), e00209–e00220. doi: 10.1128/mSphere.00209-20
Bakker J., Wangensteen O. S., Baillie C., Buddo D., Chapman D. D., Gallagher A., et al. (2019). Biodiversity assessment of tropical shelf eukaryotic communities via pelagic eDNA metabarcoding. Ecol. Evol. 9 (24), 14341–14355. doi: 10.1002/ece3.5871
Bauer J. E., Cai W. J., Raymond P. A., Bianchi T. S., Hopkinson C. S., Regnier P. A. (2013). The changing carbon cycle of the coastal ocean. Nature 504 (7478), 61–70. doi: 10.1038/nature12857
Benjamini Y., Hochberg Y. (1995). Controlling the false discovery rate: A practical and powerful approach to multiple testing. J. R. Stat. Soc.: Ser. B (Methodological) 57 (1), 289–300. doi: 10.1111/j.2517-6161.1995.tb02031.x
Bjorbækmo M., Evenstad A., Røsæg L. L., Krabberød A. K., Logares R. (2020). The planktonic protist interactome: where do we stand after a century of research? ISME J. 14 (2), 544–559. doi: 10.1038/s41396-019-0542-5
Brisson V., Schmidt J., Northen T. R., Vogel J. P., Gaudin A. (2019). A new method to correct for habitat filtering in microbial correlation networks. Front. Microbiol. 10. doi: 10.3389/fmicb.2019.00585
Brooker R. W., Maestre F. T., Callaway R. M., Lortie C. L., Cavieres L. A., Kunstler G., et al. (2008). Facilitation in plant communities: the past, the present, and the future. J. Ecol., 96 (1), 18–34, doi: 10.1111/j.1365-2745.2007.01295.x
Buchan A., LeCleir G. R., Gulvik C. A., González J. M. (2014). Master recyclers: features and functions of bacteria associated with phytoplankton blooms. Nat. Rev. Microbiol. 12 (10), 686–698. doi: 10.1038/nrmicro3326
Burki F., Sandin M. M., Jamy M. (2021). Diversity and ecology of protists revealed by metabarcoding. Curr. Biol. 31 (19), R1267–R1280. doi: 10.1016/j.cub.2021.07.066
Caron D. A., Countway P. D., Jones A. C., Kim D. Y., Schnetzer A. (2012). Marine protistan diversity. Annu. Rev. Mar. Sci. 4, 467–493. doi: 10.1146/annurev-marine-120709-142802
Cavieres L. A., Badano E. I. (2009). Do facilitative interactions increase species richness at the entire community level? J. Ecol. 97 (6), 1181–1191. doi: 10.1111/j.1365-2745.2009.01579.x
Chen W., Pan Y., Yu L., Yang J., Zhang W. (2017). Patterns and processes in marine microeukaryotic community biogeography from xiamen coastal waters and intertidal sediments, southeast China. Front. Microbiol. 8. doi: 10.3389/fmicb.2017.01912
Chow C. E., Sachdeva R., Cram J. A., Steele J. A., Needham D. M., Patel A., et al. (2013). Temporal variability and coherence of euphotic zone bacterial communities over a decade in the southern California bight. ISME J. 7 (12), 2259–2273. doi: 10.1038/ismej.2013.122
Cole J. J. (1982). Interactions between bacteria and algae in aquatic ecosystems. Annu. Rev. Ecol. Evol. Syst. 13 (1), 291–314. doi: 10.1146/annurev.es.13.110182.001451
Croft M. T., Lawrence A. D., Raux-Deery E., Warren M. J., Smith A. G. (2005). Algae acquire vitamin B12 through a symbiotic relationship with bacteria. Nature 438 (7064), 90–93. doi: 10.1038/nature04056
Cui Y., Chun S. J., Baek S. H., Lee M., Kim Y., Lee H. G., et al. (2019). The water depth-dependent co-occurrence patterns of marine bacteria in shallow and dynamic southern coast, Korea. Sci. Rep. 9 (1), 9176. doi: 10.1038/s41598-019-45512-5
de Vargas C., Audic S., Henry N., Decelle J., Mahé F., Logares R., et al. (2015). Ocean plankton. eukaryotic plankton diversity in the sunlit ocean. Sci. (New York N.Y.) 348 (6237), 1261605. doi: 10.1126/science.1261605
Durham B. P. (2021). Deciphering metabolic currencies that support marine microbial networks. mSystems 6 (4), e0076321. doi: 10.1128/mSystems.00763-21
Farnelid H., Turk-Kubo K., Zehr J. P. (2021). Cell sorting reveals few novel prokaryote and photosynthetic picoeukaryote associations in the oligotrophic ocean. Environ. Microbiol. 23 (3), 1469–1480. doi: 10.1111/1462-2920.15351
Faust K., Raes J. (2012). Microbial interactions: from networks to models. Nat. Rev. Microbiol. 10 (8), 538–550. doi: 10.1038/nrmicro2832
Fernández E., Álvarez-Salgado X. A., Beiras R., Ovejero A., Méndez G. (2016). Coexistence of urban uses and shellfish production in an upwelling-driven, highly productive marine environment: The case of the ría de vigo (Galicia, Spain). Reg. Stud. Mar. 8, 362–370. doi: 10.1016/j.rsma.2016.04.002
Fernández-Álvarez C., Santos Y. (2018). Identification and typing of fish pathogenic species of the genus tenacibaculum. Appl. Microbiol. Biotechnol. 102 (23), 9973–9989. doi: 10.1007/s00253-018-9370-1
Field C. B., Behrenfeld M. J., Randerson J. T., Falkowski P. (1998). Primary production of the biosphere: integrating terrestrial and oceanic components. Sci. (New York N.Y.) 281 (5374), 237–240. doi: 10.1126/science.281.5374.237
Forehead H., Thomson P., Kendrick G. A. (2013). Shifts in composition of microbial communities of subtidal sandy sediments zmaximize retention of nutrients. FEMS Microbiol. Ecol. 83 (2), 279–298. doi: 10.1111/j.1574-6941.2012.01472.x
Forster D., Dunthorn M., Mahé F., Dolan J. R., Audic S., Bass D., et al. (2016). Benthic protists: the under-charted majority. FEMS Microbiol. Ecol. 92 (8), fiw120. doi: 10.1093/femsec/fiw120
Fuhrman J. A. (2009). Microbial community structure and its functional implications. Nature 459 (7244), 193–199. doi: 10.1038/nature08058
Garstecki T., Wickham S., Arndt H. (2002). Effects of experimental sediment resuspension on a coastal planktonic microbial food web. Estuar. Coast. Shelf. Sci. 55 (5), 751–762. doi: 10.1006/ecss.2001.0937
Gilbert J. A., Steele J. A., Caporaso J. G., Steinbrück L., Reeder J., Temperton B., et al. (2012). Defining seasonal marine microbial community dynamics. ISME J. 6 (2), 298–308. doi: 10.1038/ismej.2011.107
Gloor G. B., Macklaim J. M., Pawlowsky-Glahn V., Egozcue J. J. (2017). Microbiome datasets are compositional: And this is not optional. Front. Microbiol. 8. doi: 10.3389/fmicb.2017.02224
Gong J., Shi F., Ma B., Dong J., Pachiadaki M., Zhang X., et al. (2015). Depth shapes α- and β-diversities of microbial eukaryotes in surficial sediments of coastal ecosystems. Environ. Microbiol. 17 (10), 3722–3737. doi: 10.1111/1462-2920.12763
Grattepanche J., Santoferrara L., Andrade J., Oliverio A., McManus G., Katz L. (2014). Distribution and diversity of oligotrich and choreotrich ciliates assessed by morphology and DGGE in temperate coastal waters. Aquat. Microb. Ecol. 71 (3), 211–221. doi: 10.3354/ame01675
Grossart H. P., Levold F., Allgaier M., Simon M., Brinkhoff T. (2005). Marine diatom species harbour distinct bacterial communities. Environ. Microbiol. 7 (6), 860–873. doi: 10.1111/j.1462-2920.2005.00759.x
Guerra A., Lens S., Rocha F. (2002). ““Impacto del hombre sobre el ecosistema de la ría de vigo: hacia una gestión integrada”,” in La ría de vigo. una aproximación integral al ecosistema marino de la ría de vigo. Eds. González-Garcés Santiso A., Vilas-Martín A. F., Álvarez-Salgado X. A. (Vigo: Instituto de Estudios Vigueses), 51–84.
Guizien K., Dupuy C., Ory P., Montanié H., Hartmann H., Chatelain M., et al. (2014). Microorganism dynamics during a rising tide: Disentangling effects of resuspension and mixing with offshore waters above an intertidal mudflat. J. Mar. Syst. 129, 178–188. doi: 10.1016/j.jmarsys.2013.05.010
Gutiérrez-Barral A., Teira E., Hernández-Ruiz M., Fernández E. (2021). Response of prokaryote community composition to riverine and atmospheric nutrients in a coastal embayment: Role of organic matter on vibrionales. Estuar. Coast. Shelf Sci. 251, 107196. doi: 10.1016/j.ecss.2021.107196
Hernández-Ruiz M., Barber-Lluch E., Prieto A., Álvarez-Salgado X. A., Logares R., Teira E. (2018). Seasonal succession of small planktonic eukaryotes inhabiting surface waters of a coastal upwelling system. Environ. Microbiol. 20 (8), 2955–2973. doi: 10.1111/1462-2920.14313
Hernando-Morales V., Varela M. M., Needham D. M., Cram J., Fuhrman J. A., Teira E. (2018). Vertical and seasonal patterns control bacterioplankton communities at two horizontally coherent coastal upwelling sites off Galicia (NW Spain). Microb. Ecol. 76 (4), 866–884. doi: 10.1007/s00248-018-1179-z
Holman L. E., de Bruyn M., Creer S., Carvalho G., Robidart J., Rius M. (2019). Detection of introduced and resident marine species using environmental DNA metabarcoding of sediment and water. Sci. Rep. 9 (1), 11559. doi: 10.1038/s41598-019-47899-7
Hoshino T., Doi H., Uramoto G. I., Wörmer L., Adhikari R. R., Xiao N., et al. (2020). Global diversity of microbial communities in marine sediment. Proc. Natl. Acad. Sci. U.S.A. 117 (44), 27587–27597. doi: 10.1073/pnas.1919139117
Hou F., Zhang H., Xie W., Zhou X., Zhu X., Zhang D. (2020). Co-Occurrence patterns and assembly processes of microeukaryotic communities in an early-spring diatom bloom. Sci. Total Environ. 711, 134624. doi: 10.1016/j.scitotenv.2019.134624
Instituto Tecnológico para el Control del Medio Marino de Galicia (INTECMAR), Unidad de Proceso de Datos y Sistemas de Informacion (2010) Estacións de mostraxe. Available at: http://www.intecmar.gal/Ctd/Default.aspx (Accessed February 21, 2022).
Itakura S., Imai I., Itoh K. (1997). ‘Seed bank’ of coastal planktonic diatoms in bottom sediments of Hiroshima bay, seto inland Sea, Japan. Mar. Biol. 128 (3), 497–508. doi: 10.1007/s002270050116
Joglar V., Álvarez-Salgado X. A., Gago-Martinez A., Leao J. M., Pérez-Martínez C., Pontiller B., et al. (2021). Cobalamin and microbial plankton dynamics along a coastal to offshore transect in the Eastern north Atlantic ocean. Environ. Microbiol. 23 (3), 1559–1583. doi: 10.1111/1462-2920.15367
Koedooder C., Stock W., Willems A., Mangelinckx S., De Troch M., Vyverman W., et al. (2019). Diatom-bacteria interactions modulate the composition and productivity of benthic diatom biofilms. Front. Microbiol. 10. doi: 10.3389/fmicb.2019.01255
Lasa A., di Cesare A., Tassistro G., Borello A., Gualdi S., Furones D., et al. (2019). Dynamics of the pacific oyster pathobiota during mortality episodes in Europe assessed by 16S rRNA gene profiling and a new target enrichment next-generation sequencing strategy. Environ. Microbiol. 21 (12), 4548–4562. doi: 10.1111/1462-2920.14750
Leray M., Knowlton N. (2016). Censusing marine eukaryotic diversity in the twenty-first century. Philos. Trans. R. Soc Lond. B Biol. Sci. 3711702, 20150331. doi: 10.1098/rstb.2015.0331
Levin L. A., Boesch D. F., Covich A., Dahm C., Erséus C., Ewel K. C., et al. (2001). The function of marine critical transition zones and the importance of sediment biodiversity. Ecosystems 4 (5), 430–451. doi: 10.1007/s10021-001-0021-4
Lex A., Gehlenborg N., Strobelt H., Vuillemot R., Pfister H. (2014). UpSet: Visualization of intersecting sets. IEEE Trans. Vis. Comput. Graph. 20 (12), 1983–1992. doi: 10.1109/TVCG.2014.2346248
Li L., Kato C., Horikoshi K. (1999). Bacterial diversity in deep-sea sediments from different depths. Biodivers. Conserv. 8, 659–677. doi: 10.1023/A:1008848203739
Likumahua S., Sangiorgi F., de Boer M. K., Tatipatta W. M., Pelasula D. D., Polnaya D., et al. (2021). Dinoflagellate cyst distribution in surface sediments of ambon bay (eastern indonesia): Environmental conditions and harmful blooms. Mar. pollut. Bull. 166, 112269. doi: 10.1016/j.marpolbul.2021.112269
Liu Q., Zhao Q., Jiang Y., Li Y., Zhang C., Li X., et al. (2021). Diversity and co-occurrence networks of picoeukaryotes as a tool for indicating underlying environmental heterogeneity in the Western pacific ocean. Mar. Environ. Res. 170, 105376. doi: 10.1016/j.marenvres.2021.105376
Loreau M., Hector A. (2001). Partitioning selection and complementarity in biodiversity experiments. Nature 4126842, 72–76. doi: 10.1038/35083573
Luria C. M., Amaral-Zettler L. A., Ducklow H. W., Rich J. J. (2016). Seasonal succession of free-living bacterial communities in coastal waters of the Western Antarctic peninsula. Front. Microbiol. 7. doi: 10.3389/fmicb.2016.01731
Mantel N. (1967). The detection of disease clustering and a generalized regression approach. Cancer Res. 27 (2), 209–220.
Martinez-Garcia M., Brazel D., Poulton N. J., Swan B. K., Gomez M. L., Masland D., et al. (2012). Unveiling in situ interactions between marine protists and bacteria through single cell sequencing. ISME J. 6 (3), 703–707. doi: 10.1038/ismej.2011.126
Massana R. (2011). Eukaryotic picoplankton in surface oceans. Annu. Rev. Microbiol. 65, 91–110. doi: 10.1146/annurev-micro-090110-102903
Massana R., Gobet A., Audic S., Bass D., Bittner L., Boutte C., et al. (2015). Marine protist diversity in European coastal waters and sediments as revealed by high-throughput sequencing. Environ. Microbiol. 17 (10), 4035–4049. doi: 10.1111/1462-2920.12955
Mestre M., Borrull E., Sala M., Gasol J. M. (2017). Patterns of bacterial diversity in the marine planktonic particulate matter continuum. ISME J. 11 (4), 999–1010. doi: 10.1038/ismej.2016.166
MeteoGalicia, Xunta de Galicia (2022) Histórico da rede meteorolóxica. Available at: https://www.meteogalicia.gal/observacion/estacionshistorico/historico.action?idEst=14001 (Accessed February 21, 2022).
Middelboe M., Brussaard C. (2017). Marine viruses: Key players in marine ecosystems. Viruses 9 (10), 302. doi: 10.3390/v9100302
Milici M., Deng Z. L., Tomasch J., Decelle J., Wos-Oxley M. L., Wang H., et al. (2016). Co-Occurrence analysis of microbial taxa in the Atlantic ocean reveals high connectivity in the free-living bacterioplankton. Front. Microbiol. 7. doi: 10.3389/fmicb.2016.00649
Molino P. J., Wetherbee R. (2008). The biology of biofouling diatoms and their role in the development of microbial slimes. Biofouling 24 (5), 365–379. doi: 10.1080/08927010802254583
Needham D. M., Fuhrman J. A. (2016). Pronounced daily succession of phytoplankton, archaea and bacteria following a spring bloom. Nat. Microbiol. 1, 16005. doi: 10.1038/nmicrobiol.2016.5
O'Donnell J. L., Kelly R. P., Shelton A. O., Samhouri J. F., Lowell N. C., Williams G. D. (2017). Spatial distribution of environmental DNA in a nearshore marine habitat. PeerJ 5, e3044. doi: 10.7717/peerj.3044
Pavan-Kumar A., Gireesh-Babu P., Lakra W. S. (2015). DNA Metabarcoding: A new approach for rapid biodiversity assessment. J. Cell. Sci. Mol. Biol. 2, 111.
Pecl G. T., Araújo M. B., Bell J. D., Blanchard J., Bonebrake T. C., Chen I. C., et al. (2017). Biodiversity redistribution under climate change: Impacts on ecosystems and human well-being. Sci. (New York N.Y.) 355 (6332), eaai9214. doi: 10.1126/science.aai9214
Perkins T. L., Clements K., Baas J. H., Jago C. F., Jones D. L., Malham S. K., et al. (2014). Sediment composition influences spatial variation in the abundance of human pathogen indicator bacteria within an estuarine environment. PloS One 9 (11), e112951. doi: 10.1371/journal.pone.0112951
Peters J. A., Lodge D. M. (2009). “Littoral zone” in The encyclopedia of inland waters, vol. vol.1 . Ed. Likens G. E. (Oxford: Elsevier), 79–87.
Probandt D., Knittel K., Tegetmeyer H. E., Ahmerkamp S., Holtappels M., Amann R. (2017). Permeability shapes bacterial communities in sublittoral surface sediments. Environ. Microbiol. 19 (4), 1584–1599. doi: 10.1111/1462-2920.13676
Ray G. L. (2005). “Ecological functions of shallow, unvegetated estuarine habitats and potential dredging impacts (with emphasis on Chesapeake bay),” in WRAP technical notes collection (ERDC TN-WRAP-05-3), U.S. army engineer research and development center (Vicksburg, MS). Available at: http://el.erdc.usace.army.mil/wrap.
Ríos-Castro R., Romero A., Aranguren R., Pallavicini A., Banchi E., Novoa B., et al. (2021). High-throughput sequencing of environmental DNA as a tool for monitoring eukaryotic communities and potential pathogens in a coastal upwelling ecosystem. Front. Vet. Sci. 8. doi: 10.3389/fvets.2021.765606
Rodríguez-Martínez J. M., Pascual A. (2006). Antimicrobial resistance in bacterial biofilms. Rev. Med. Microbiol. 17 (3), 65–75. doi: 10.1097/01.revmedmi.0000259645.20603.63
Salazar G., Cornejo-Castillo F. M., Benítez-Barrios V., Fraile-Nuez E., Álvarez-Salgado X. A., Duarte C. M., et al. (2016). Global diversity and biogeography of deep-sea pelagic prokaryotes. ISME J. 10 (3), 596–608. doi: 10.1038/ismej.2015.137
Salazar G., Sunagawa S. (2017). Marine microbial diversity. Curr. Biol. 27 (11), R489–R494. doi: 10.1016/j.cub.2017.01.017
Sánchez-Soto Jiménez M. F., Cerqueda-García D., Montero-Muñoz J. L., Aguirre-Macedo M. L., García-Maldonado J. Q. (2018). Assessment of the bacterial community structure in shallow and deep sediments of the perdido fold belt region in the gulf of Mexico. PeerJ 6, e5583. doi: 10.7717/peerj.5583
Sapp M., Schwaderer A. S., Wiltshire K. H., Hoppe H. G., Gerdts G., Wichels A. (2007). Species-specific bacterial communities in the phycosphere of microalgae? Microb. Ecol. 53 (4), 683–699. doi: 10.1007/s00248-006-9162-5
Serôdio J., Lavaud J. (2020). “Diatoms and their ecological importance” in Life below water, encyclopedia of the UN sustainable development goals. Eds. Filho W. L., Azul A. M., Brandli L., Salvia A. L., Wall T. (Cham: Springer), 1–9. doi: 10.1007/978-3-319-71064-8_12-1.hal-03113866
Sheu S. Y., Lin K. Y., Chou J. H., Chang P. S., Arun A. B., Young C. C., et al. (2007). Tenacibaculum litopenaei sp. nov., isolated from a shrimp mariculture pond. Int. J. Syst. Evol. 57 (Pt 5), 1148–1153. doi: 10.1099/ijs.0.64920-0
Sieburth J. M., Smetacek V., Lenz J. (1978). IPelagic ecosystem structure: Heterotrophic compartments of the plankton and their relationship to plankton size fractions 1. Limnol Oceanography 23 (6), 1256–1263. doi: 10.4319/lo.1978.23.6.1256
Simon M., Jardillier L., Deschamps P., Moreira D., Restoux G., Bertolino P., et al. (2015). Complex communities of small protists and unexpected occurrence of typical marine lineages in shallow freshwater systems. Environ. Microbiol. 17 (10), 3610–3627. doi: 10.1111/1462-2920.12591
Solden L., Lloyd K., Wrighton K. (2016). The bright side of microbial dark matter: lessons learned from the uncultivated majority. Curr. Opin. Microbiol. 31, 217–226. doi: 10.1016/j.mib.2016.04.020
Steele J. A., Countway P. D., Xia L., Vigil P. D., Beman J. M., Kim D. Y., et al. (2011). Marine bacterial, archaeal and protistan association networks reveal ecological linkages. ISME J. 5 (9), 1414–1425. doi: 10.1038/ismej.2011.24
Stock W., Blommaert L., De Troch M., Mangelinckx S., Willems A., Vyverman W., et al. (2019). Host specificity in diatom-bacteria interactions alleviates antagonistic effects. FEMS Microbiol. Ecol. 95 (11), fiz171. doi: 10.1093/femsec/fiz171
Turner A. D., Lewis A. M., Bradley K., Maskrey B. H. (2021). Marine invertebrate interactions with harmful algal blooms - implications for one health. J. Invertebr. Pathol. 186, 107555. doi: 10.1016/j.jip.2021.107555
Ul-Hasan S., Bowers R. M., Figueroa-Montiel A., Licea-Navarro A. F., Beman J. M., Woyke T., et al. (2019). Community ecology across bacteria, archaea and microbial eukaryotes in the sediment and seawater of coastal Puerto nuevo, Baja California. PloS One 14 (2), e0212355. doi: 10.1371/journal.pone.0212355
Vanelslander B., de Wever A., van Oostende N., Kaewnuratchadasorn P., Vanormelingen P., Hendrickx F., et al. (2009). Complementarity effects drive positive diversity effects on biomass production in experimental benthic diatom biofilms. J. Ecol. 97 (5), 1075–1082. doi: 10.1111/j.1365-2745.2009.01535.x
Veilleux H. D., Misutka M. D., Glover C. N. (2021). Environmental DNA and environmental RNA: Current and prospective applications for biological monitoring. Sci. Total Environ. 782, 146891. doi: 10.1016/j.scitotenv.2021.146891
Whittaker R. H. (1969). New concepts of kingdoms or organisms. evolutionary relations are better represented by new classifications than by the traditional two kingdoms. Sci. (New York N.Y.) 163 (3863), 150–160. doi: 10.1126/science.163.3863.150
Wichard T., Gerecht A., Boersma M., Poulet S. A., Wiltshire K., Pohnert G. (2007). Lipid and fatty acid composition of diatoms revisited: rapid wound-activated change of food quality parameters influences herbivorous copepod reproductive success. ChemBioChem 8 (10), 1146–1153. doi: 10.1002/cbic.200700053
Yu X., Li X., Liu Q., Yang M., Wang X., Guan Z., et al. (2022). Community assembly and co-occurrence network complexity of pelagic ciliates in response to environmental heterogeneity affected by sea ice melting in the Ross Sea, Antarctica. Sci. total Environ. 836, 155695. doi: 10.1016/j.scitotenv.2022.155695
Zhao F., Wang C., Xu K., Huang P., Zhou T. (2020). Diversity and connectivity of microeukaryote communities across multiple habitats from intertidal zone to deep-sea floor in the Western pacific ocean. Deep Sea Res. Part I: Oceanogr. Res. Pap. 165, 103395. doi: 10.1016/j.dsr.2020.103395
Zheng Q., Wang Y., Lu J., Lin W., Chen F., Jiao N. (2020). Metagenomic and metaproteomic insights into photoautotrophic and heterotrophic interactions in a synechococcus culture. mBio 11 (1), e03261–e03219. doi: 10.1128/mBio.03261-19
Zhou L., Chen C., Xie J., Xu C., Zhao Q., Qin J. G., et al. (2019). Intestinal bacterial signatures of the "cotton shrimp-like" disease explain the change of growth performance and immune responses in pacific white shrimp (Litopenaeus vannamei). Fish Shellfish Immunol. 92, 629–636. doi: 10.1016/j.fsi.2019.06.054
Zhou J., Richlen M. L., Sehein T. R., Kulis D. M., Anderson D. M., Cai Z. (2018). Microbial community structure and associations during a marine dinoflagellate bloom. Front. Microbiol. 9. doi: 10.3389/fmicb.2018.01201
Keywords: eukaryote, prokaryote, benthonic, planktonic, co-occurrence network, metabarcoding, shallow marine ecosystem
Citation: Ríos-Castro R, Costas-Selas C, Pallavicini A, Vezzulli L, Novoa B, Teira E and Figueras A (2022) Co-occurrence and diversity patterns of benthonic and planktonic communities in a shallow marine ecosystem. Front. Mar. Sci. 9:934976. doi: 10.3389/fmars.2022.934976
Received: 03 May 2022; Accepted: 29 July 2022;
Published: 23 August 2022.
Edited by:
Michaela M. Salcher, Academy of Sciences of the Czech Republic (ASCR), CzechiaReviewed by:
Feng Zhao, Institute of Oceanology (CAS), ChinaIrini Tsikopoulou, Hellenic Center for Marine Research, Greece
Copyright © 2022 Ríos-Castro, Costas-Selas, Pallavicini, Vezzulli, Novoa, Teira and Figueras. This is an open-access article distributed under the terms of the Creative Commons Attribution License (CC BY). The use, distribution or reproduction in other forums is permitted, provided the original author(s) and the copyright owner(s) are credited and that the original publication in this journal is cited, in accordance with accepted academic practice. No use, distribution or reproduction is permitted which does not comply with these terms.
*Correspondence: Antonio Figueras, antoniofigueras@iim.csic.es; Eva Teira, teira@uvigo.es
†These authors have contributed equally to this work